Probability
Explore the Flashcards:
The field of probability, within mathematics, assesses the chances of an event occurring.
This likelihood is represented by a numerical value ranging between 0 and 1, where higher values indicate a greater probability.
Event
Events are sets of outcomes in experiments with assigned probabilities. A single outcome can belong to many different events,
which are not equally likely since they can include different groups of outcomes.
Likelihood
A measure of how well a statistical model explains the observed data. In probability,
it often refers to the probability of an interval rather than an individual value.
Outcome
A single result from a probability experiment. Each distinct outcome has a probability assigned to it based on certain criteria,
such as the size of its associated interval in a probability distribution.
Preferred Outcomes
Preferred outcomes are the outcomes we want to occur or the outcomes we are interested in. We also call refer to such
outcomes as “Favorable”.
Sample Space
Sample space refers to all possible outcomes that can occur. Its “size” indicates the amount of elements in it.
Probability Formula
The Probability of event X occurring equals the number of preferred outcomes over the number of outcomes in the
sample space.
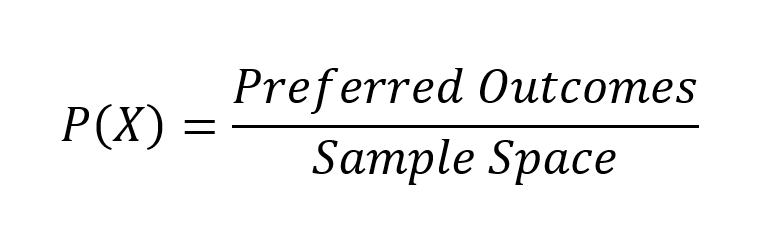
Trial
Observing an event occur and recording the outcome.
Experiment
A collection of one or multiple trials.
Experimental Probability
The probability we assign an event, based on an experiment we conduct.
Expected Value
The weighted average of all possible values that a random variable can take on.
It is the specific outcome we expect to occur when we run an experiment.
Expected value for categorical variables.
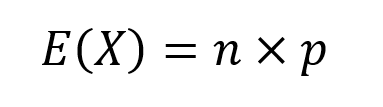
Expected Value (Numerical Variables)
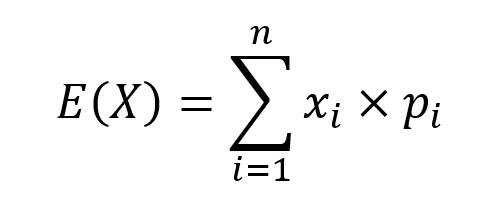
Frequency
Frequency is the number of times a given value or outcome appears in the sample space.
Frequency Distribution Table
The frequency distribution table is a table matching each distinct outcome in the sample space to its associated frequency.
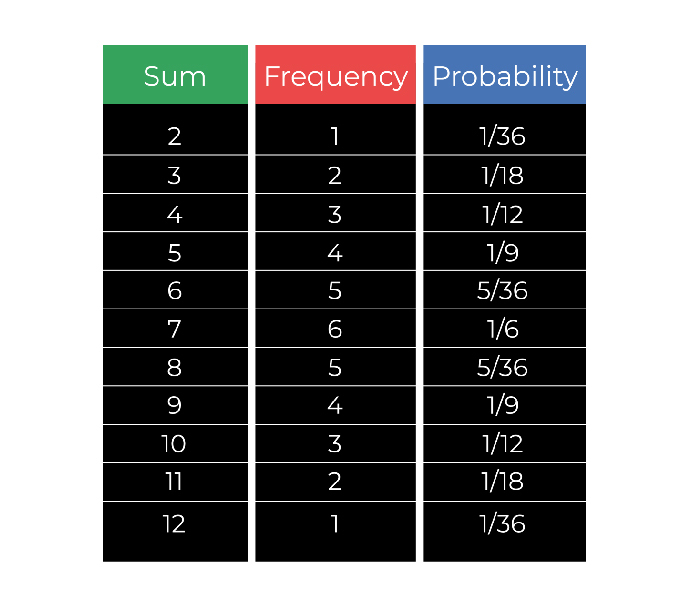
Probability Frequency Distribution
A collection of the probabilities for each possible outcome of an event.
Complement
The complement of an event is everything an event is not.
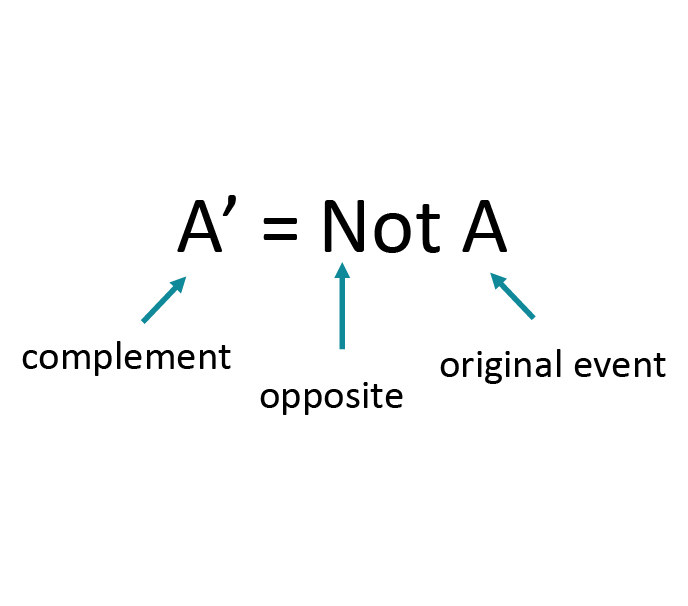
Characteristics of Complements
• Can never occur simultaneously.
• Add up to the sample space. (A + A’ = Sample space)
• Their probabilities add up to 1. (P(A) + P(A’) = 1)
• The complement of a complement is the original event. ((A’)’ = A)
The probability of an event A is 0.25.
What is the probability of A’ (the compliment of A)?
P(A’) = 0.75
Combinatorics
A branch of mathematics dealing with combinations of objects belonging to a finite set in accordance with certain constraints.
Factorials
Factorials express the product of all integers from 1 to n and we denote them with the “!” symbol.

Key Values for Factorials
• 0! = 1.
• If n<0, n! does not exist.
Rules for Factorial Multiplication. (For n>0 and n>k)
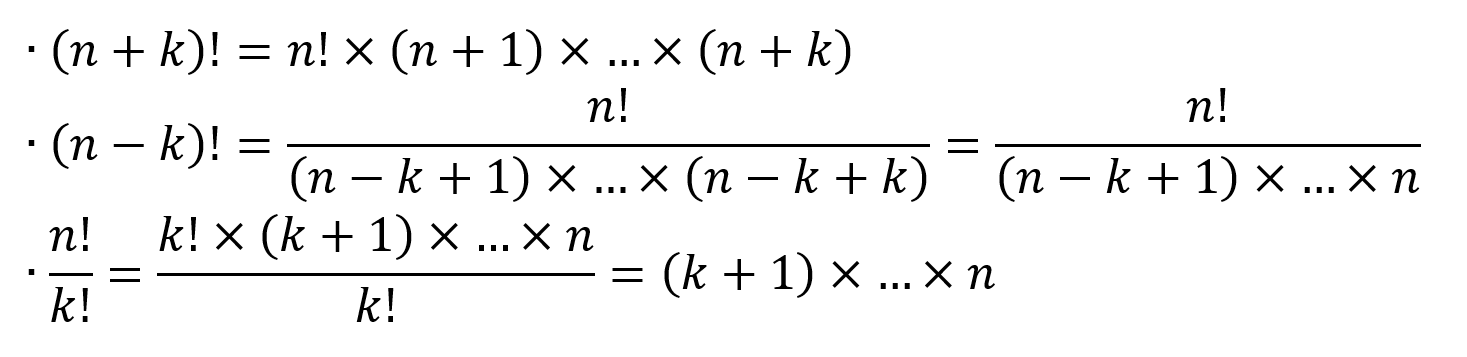
Natural Numbers
The set of positive integers (1, 2, 3, ...) that are used in counting. Factorials are computed using these numbers.
Permutations
Permutations represent the number of different possible ways we can arrange a number of elements.
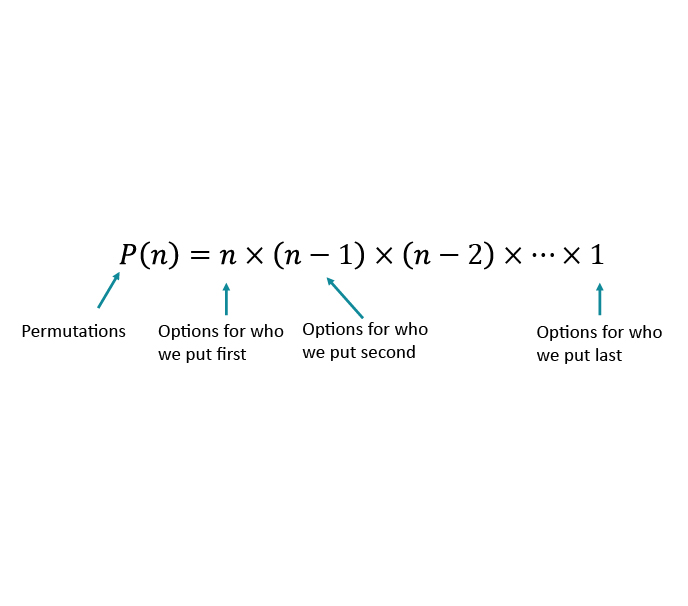
Characteristics of Permutations
• Arranging all elements within the sample space.
• No repetition.
• Pn = n × (n − 1) × (n − 2) × ⋯ × 1 = n! (Called “n factorial”)
In how many ways can we arrange 5 people?
120
Variations
Variations represent the number of different possible ways we can pick and arrange a number of elements.
Variations (With Repetition)
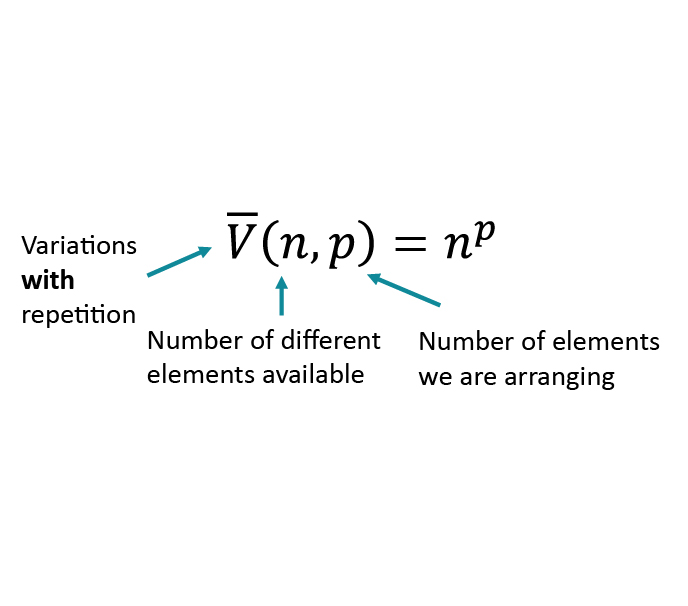
Variations (Without Repetition)
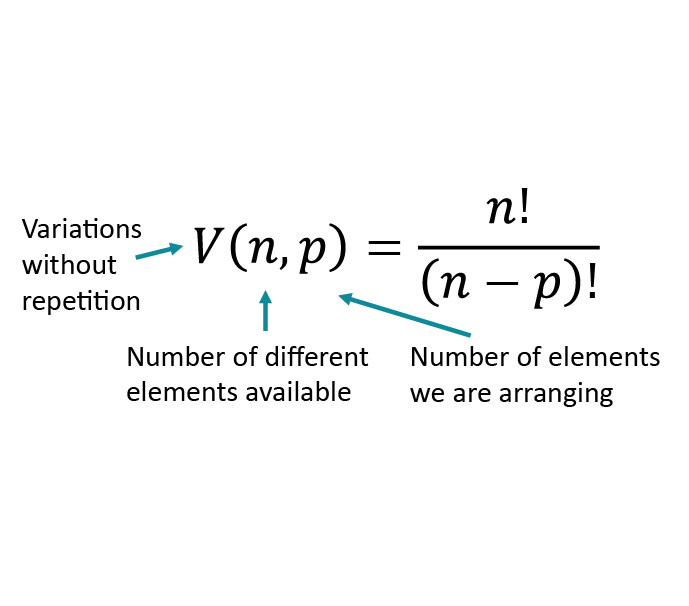
Combinations
Combinations represent the number of different possible ways we can pick a number of elements.
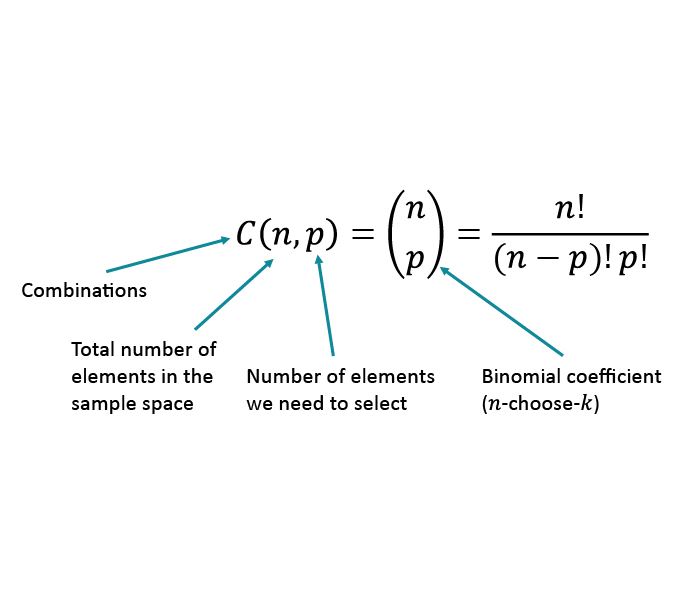
Characteristics of Combinations
• Takes into account double-counting.
• All the different permutations of a single combination are different variations.
• Combinations are symmetric, so C(p, n) = C((n−p), n) , since selecting p elements is the same as omitting n-p elements.
Combinations (Separate Sample Space)
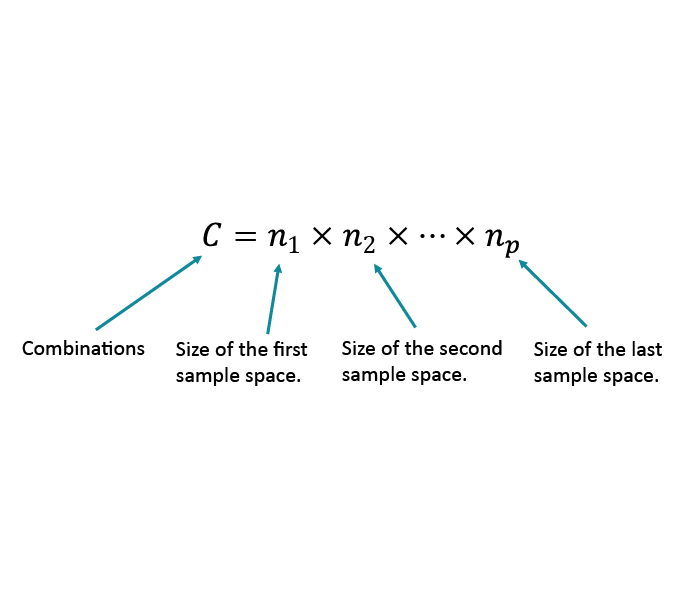
Characteristics of Combinations (Separate Sample Space)
• The option we choose for any element does not affect the number of options for the other elements.
• The order in which we pick the individual elements is arbitrary.
• We need to know the size of the sample space for each individual element.
Combinations with Repetition
Combinations represent the number of different possible ways we can pick a number of elements. In special
cases we can have repetition in combinations and for those we use a different formula.
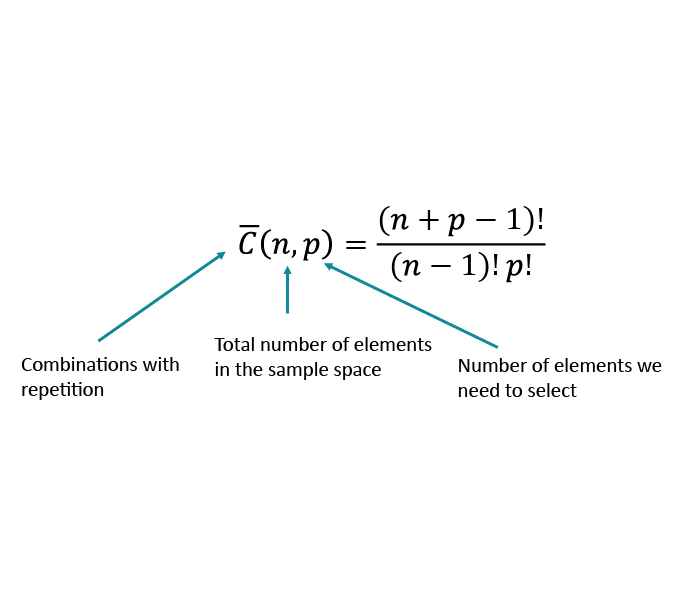
Set
A set is a collection of elements, which hold certain values. Additionally, every event has a set of outcomes that satisfy it.
The Null-Set
The null-set (or empty set), denoted ∅, is an set which contain no values.
Finite Set
A set with a limited number of elements, which is a fundamental concept in combinatorics.
𝑥 ∈ A
Element x is a part of set A.
A ∋ 𝑥
Set A contains element x.
𝑥 ∉ 𝐴
Element x is NOT a part of set A.
∀𝑥:
For all/any x such that…
A ⊆ B
A is a subset of B.
Intersection (A ∩ B)
The intersection of two or more events expresses the set of outcomes that satisfy all the events simultaneously.
Graphically, this is the area where the sets intersect.
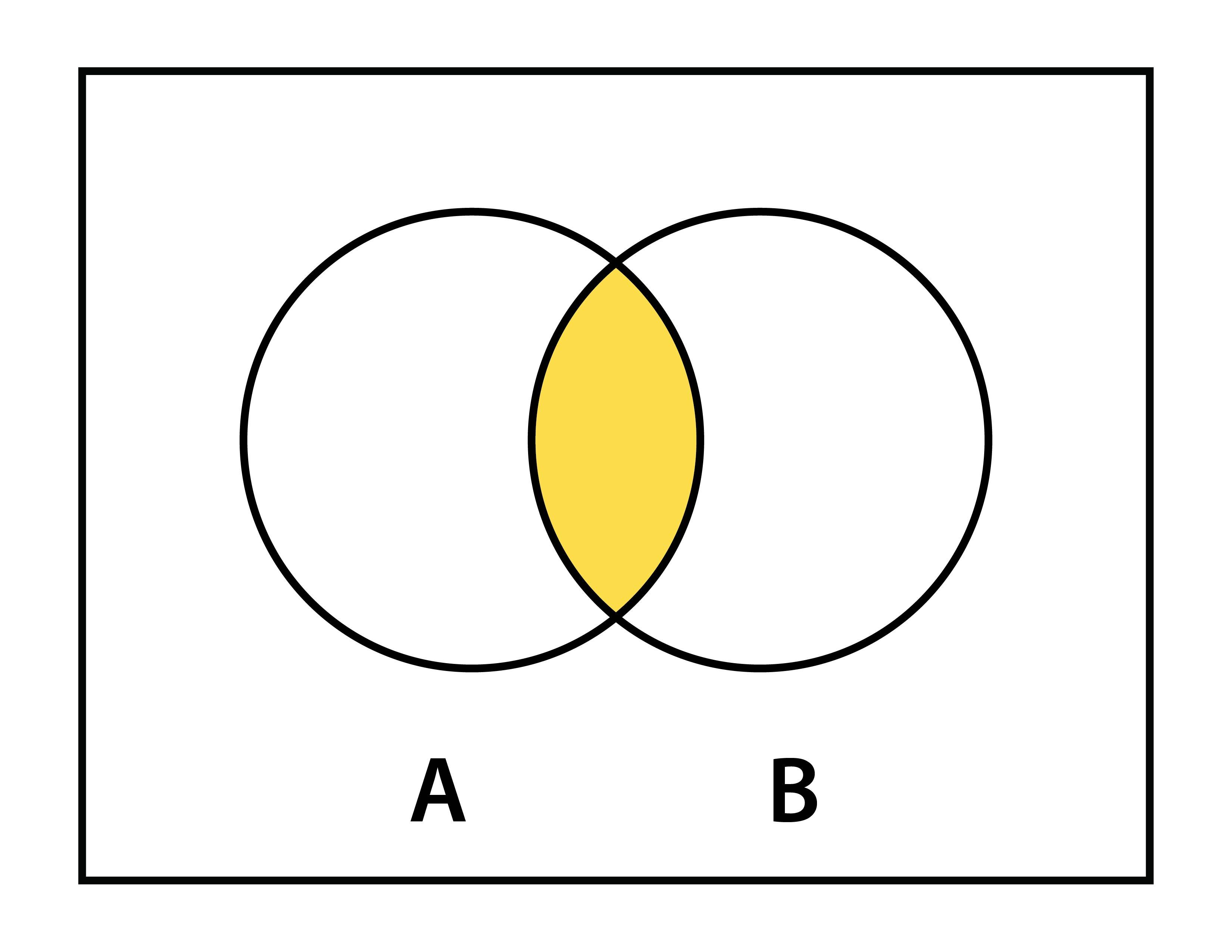
Union (A ∪ B)
The union of two or more events expresses the set of outcomes that satisfy at least one of the events.
Graphically, this is the area that includes both sets.
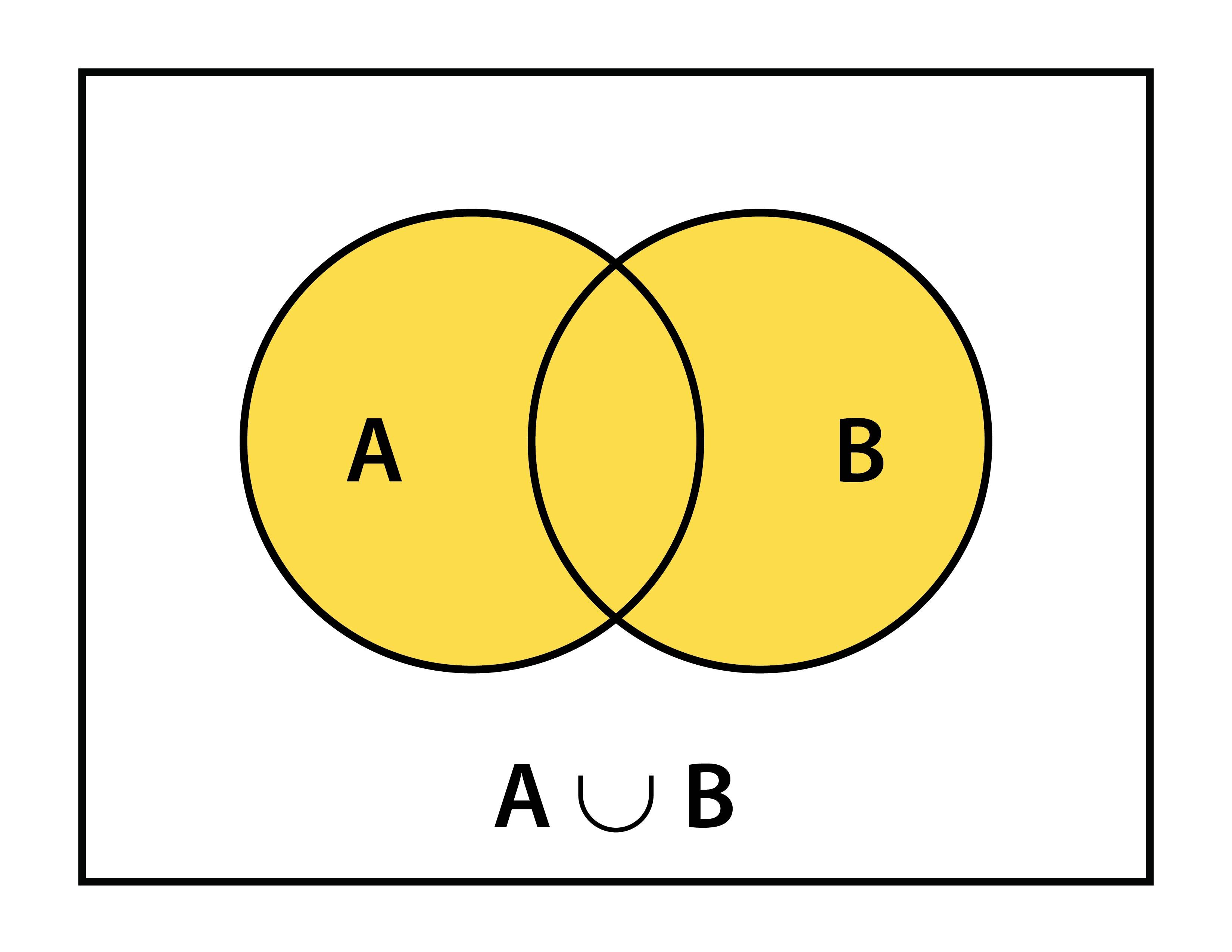
Union Formula

Mutually Exclusive Sets
Sets with no overlapping elements (A ∩ B = ∅) are called mutually exclusive. Graphically, their circles never touch.
Independent Events
If two events are independent, the probability of them occurring simultaneously equals the product of them occurring on their own.
P(A∩B) = P(A) × P(B).
Dependent Events
Two or more events in which the outcome of one event affects the outcome of the other(s). Mathematically, events A and B are dependent if and only if: P(A∩B) ≠ P(A) × P(B).
Conditional Probability
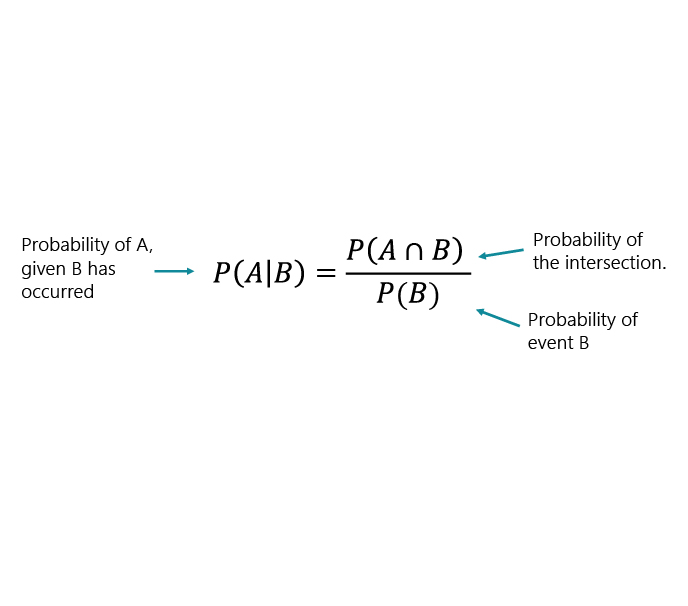
P(A|B)
The probability of event A occurring given that event B has occurred.
P(B|A)
The probability of event B occurring given that event A has occurred.
Law of Total Probability
The law of total probability dictates that for any set A,
which is a union of many mutually exclusive sets B1,B2, … , Bn, its probability equals the following sum.
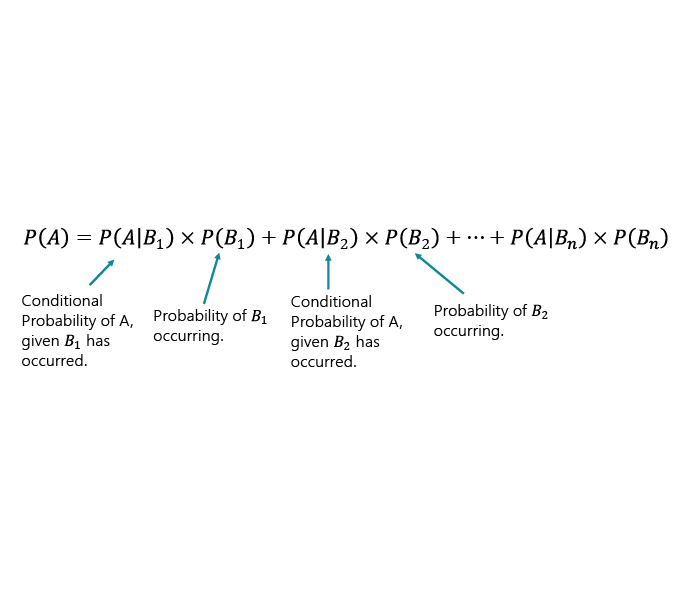
Additive Law
The additive law calculates the probability of the union based on the probability of the individual sets it accounts for.
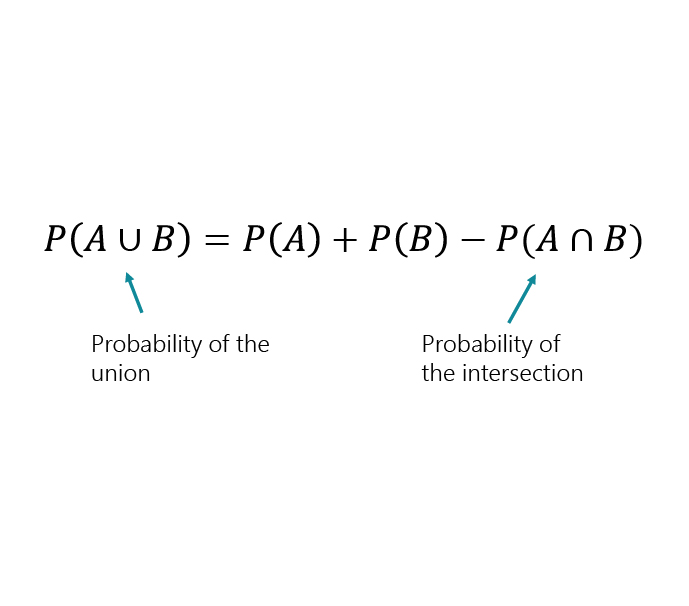
The Multiplication Rule
The multiplication rule calculates the probability of the intersection based on the conditional probability.
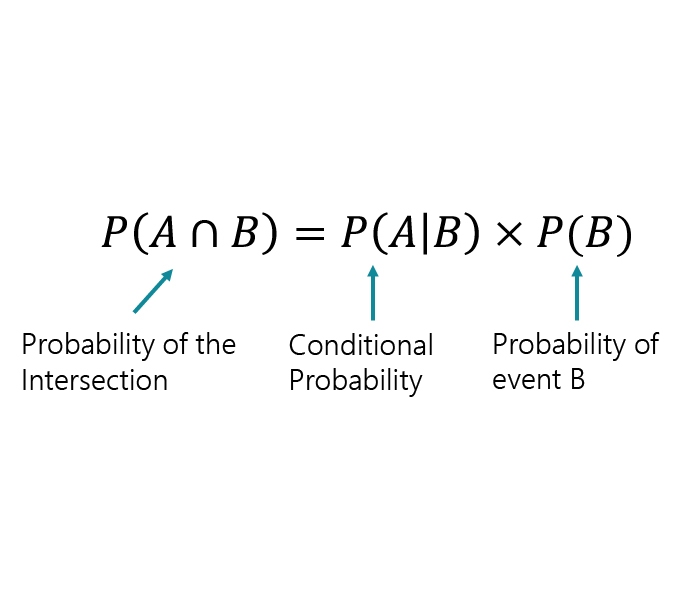
Bayes' Law
Bayes’ Law helps us understand the relationship between two events by computing the different conditional probabilities.
We also call it Bayes’ Rule or Bayes’ Theorem.
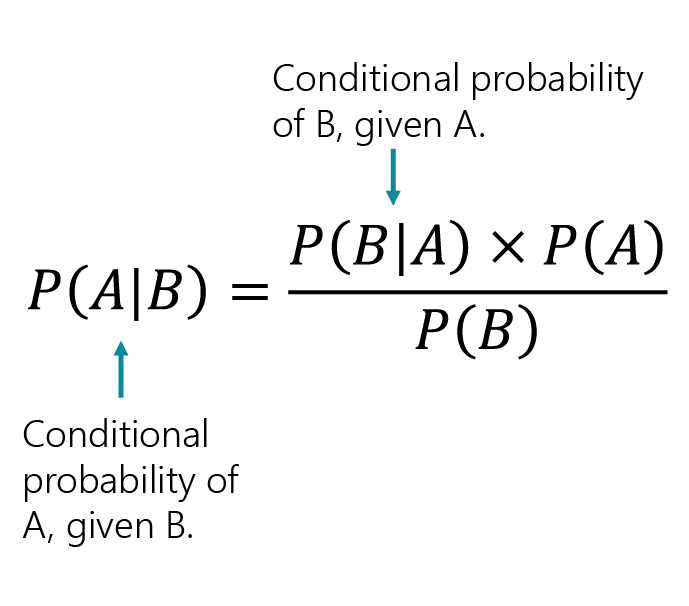
Distribution
A distribution shows the possible values a random variable can take and how frequently they occur.
Probability Function
A function that assigns a probability to each distinct outcome
in the sample space. P(Y = y), equivalent to p(y), where Y is the actual outcome and y is one of the possible outcomes.
Discrete Distributions
• Have a finite number of outcomes.
• Can add up individual values to determine
probability of an interval.
• Expected Values might be unattainable.
• Graph consists of bars lined up one after the
other.
Continuous Distributions
• Have infinitely many consecutive possible
values.
• Cannot add up the individual values that make
up an interval because there are infinitely
many of them.
• Graph consists of a smooth curve.
Variance
A measure of how far a set of numbers are spread out from their average value.
Standard Deviation
A measure of the amount of variation or dispersion of a set of values, calculated as the square root of the variance.
Uniform Distribution (Y ~ U(a, b))
A distribution where all the outcomes are equally likely is called a Uniform Distribution.
Bernoulli Distribution (Y ~ Bern(p))
A distribution consisting of a single trial and only two possible outcomes – success or failure is called a Bernoulli Distribution.
• E(Y) = p.
• Var(Y) = p × (1 − p).
Binomial Distribution (Y~ B(n, p))
A sequence of identical Bernoulli events is called Binomial and follows a Binomial Distribution.
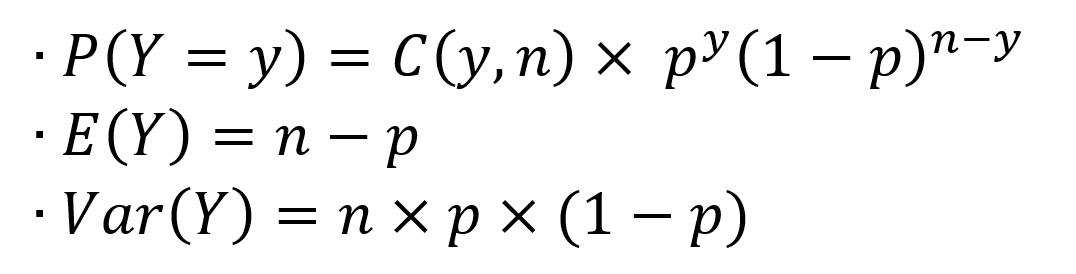
Poisson Distribution (Y~ Po(λ))
When we want to know the likelihood of a certain event occurring over a given interval of time or distance, we use a Poisson Distribution.
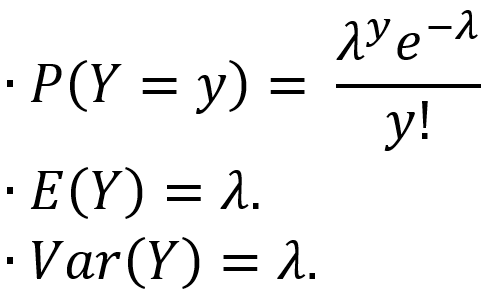
Euler’s Number (e)
A mathematical constant approximately equal to 2.72, used in various mathematical calculations, including the Poisson Distribution.

A Normal Distribution represents a distribution that most natural events follow.

Mean (μ)
The average value of a set of numbers, used as a parameter to define a Normal Distribution.
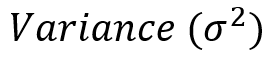
A measure of how spread out the values in a data set are, used as a parameter to define a Normal Distribution.
Symmetry in Normal Distribution
The property of a Normal Distribution where the left and right sides of the graph are mirror images of each other.
Bell-Shaped Curve
The characteristic shape of the graph of a Normal Distribution.
Outliers
Data points that fall far outside the majority of the other points in a data set.
68-95-99.7 Rule
A rule that describes the percentage of data within 1, 2, and 3 standard deviations of the mean in a Normal Distribution (approximately 68%, 95%, and 99.7%, respectively).
Standardizing a Normal Distribution
To standardize any normal distribution we need to transform it so that the mean is 0 and the variance and standard deviation are 1.
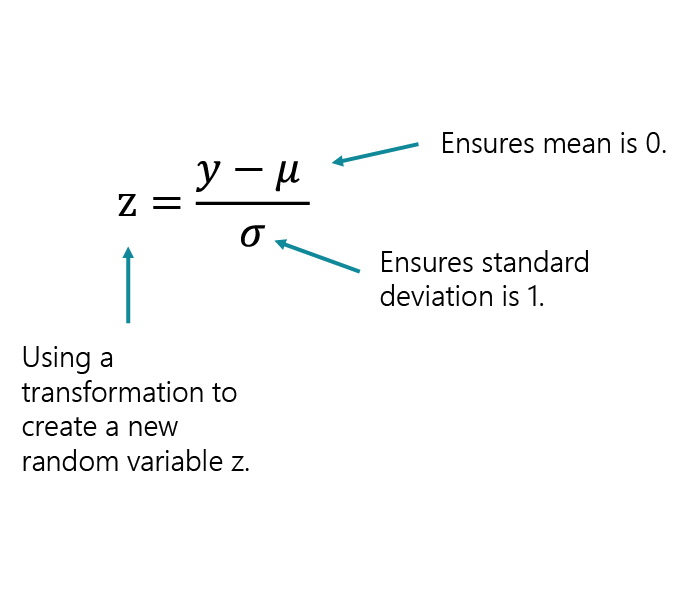
Students’ T Distribution (Y~ t(k))
Represents a small sample size approximation of a Normal Distribution.


• Its graph is asymmetric and skewed to the right.
• E(Y)= k.
• Var(Y) = 2k.
• The Chi-Squared distribution is the square of the t-distribution.
Scale Parameter
The rate parameter of an exponential distribution that determines the shape of its probability distribution function (λ).
Exponential Distribution (Y ~ Exp(λ))
The Exponential Distribution is usually observed in events which significantly change early on.
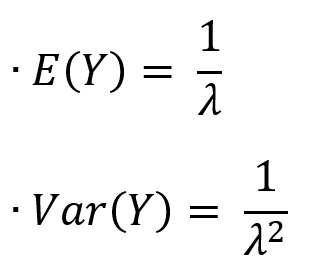
Location Parameter
In the context of the logistic distribution, it represents the mean of the distribution and is synonymous with the location.
Logistic Distribution (Y~ Logstic(μ, s))
The Continuous Logistic Distribution is observed when trying to determine how continuous variable inputs can affect the probability of a binary outcome.
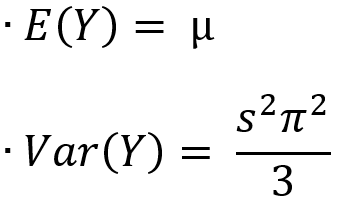
Correlation
A statistical measure that describes the extent to which two variables are related and change together.
Categorical Outcomes
Outcomes that can be placed into distinct categories but do not have an inherent order or numerical value.
Numerical Outcomes
Outcomes that are represented by numbers and can be placed in numerical order.
Option Pricing
A fundamental concept in financial mathematics, option pricing involves calculating the fair value of options (financial derivatives), using models that incorporate various factors and probabilistic assumptions.
Financial Derivatives
Financial securities whose value is dependent on or derived from an underlying asset or group of assets.
Risk Assessment
The process of identifying, analyzing, and accepting or mitigating uncertainty in investment decisions.
Uncertain Future Events
Refers to future occurrences in the financial world that cannot be predicted with certainty, necessitating the use of probability and statistical models to estimate outcomes.