18.07.2025
Data Cleaning and Preprocessing with pandas
with
Martin Ganchev
Master Python’s quintessential pandas library and its core data structures – Series and DataFrame objects. Elevate your data analysis skills for real-world challenges
3 hours of content
18961 students
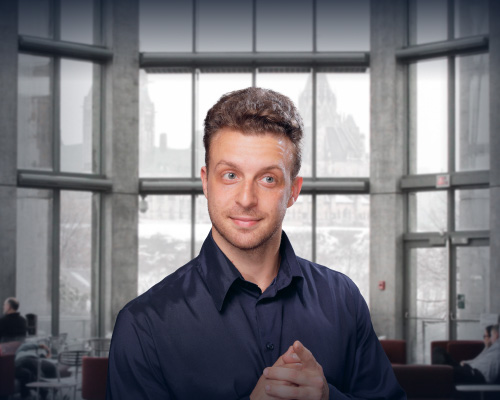
What you get:
- 3 hours of content
- 23 Interactive exercises
- 9 Coding exercises
- 18 Downloadable resources
- World-class instructor
- Closed captions
- Q&A support
- Future course updates
- Course exam
- Certificate of achievement
Data Cleaning and Preprocessing with pandas
A course by
Martin Ganchev
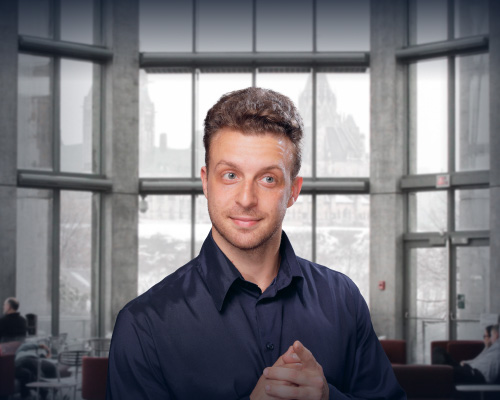
What you get:
- 3 hours of content
- 23 Interactive exercises
- 9 Coding exercises
- 18 Downloadable resources
- World-class instructor
- Closed captions
- Q&A support
- Future course updates
- Course exam
- Certificate of achievement
$99.00
Lifetime access
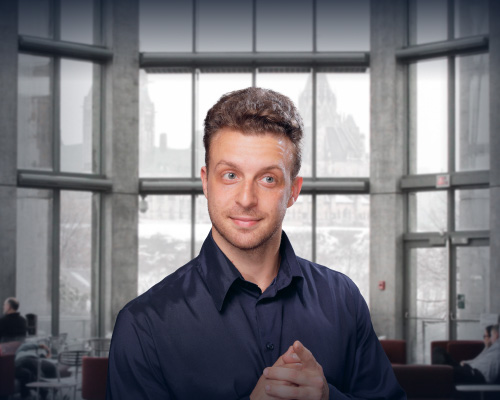
What you get:
- 3 hours of content
- 23 Interactive exercises
- 9 Coding exercises
- 18 Downloadable resources
- World-class instructor
- Closed captions
- Q&A support
- Future course updates
- Course exam
- Certificate of achievement
What You Learn
- Add the popular pandas library to your data analysis skillset to enhance your capabilities
- Learn how to install and import Python packages
- Gain proficiency in using pandas’ series and DataFrame objects to enhance your data analysis skillset
- Explore and master different ways to clean and preprocess data in pandas
- Solve real-world data preprocessing problems with pandas
- Elevate your career with advanced pandas skills, making your resume stand out to recruiters and hiring managers
Top Choice of Leading Companies Worldwide
Industry leaders and professionals globally rely on this top-rated course to enhance their skills.
Course Description
pandas is one of today’s most celebrated data analysis libraries. A favorite of many, its versatile functionalities can be leveraged for manipulation of many types of data - numeric, text, Boolean, and more. That’s one of the features that make pandas the go-to choice for analysts, especially during the data cleaning and preprocessing stages. pandas is built on NumPy and takes advantage of its computational power and abilities. But what sets pandas apart is its ability to operate with data in an easy-to-use way, allowing you to focus almost entirely on your analytic task. In this course, you will learn how to work with this powerful Python library and its core data structures – the pandas Series and DataFrames.
Learn for Free
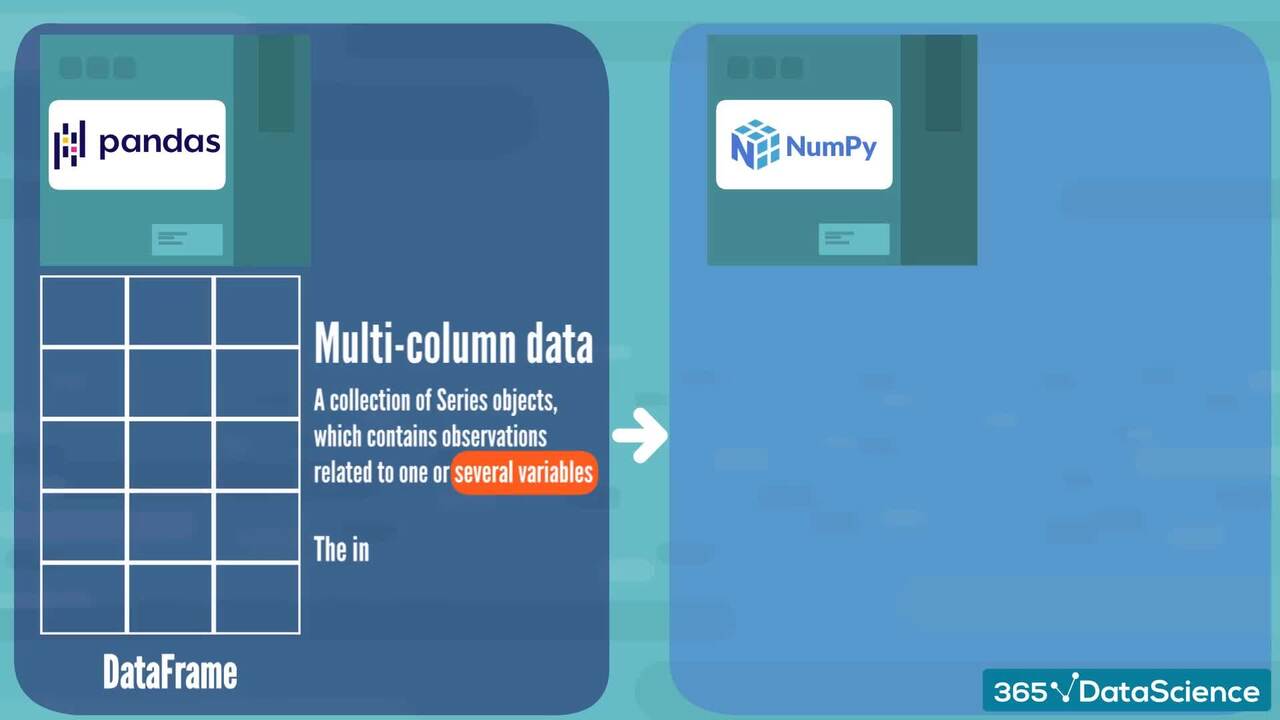
1.1 Introduction to the pandas Library
6 min
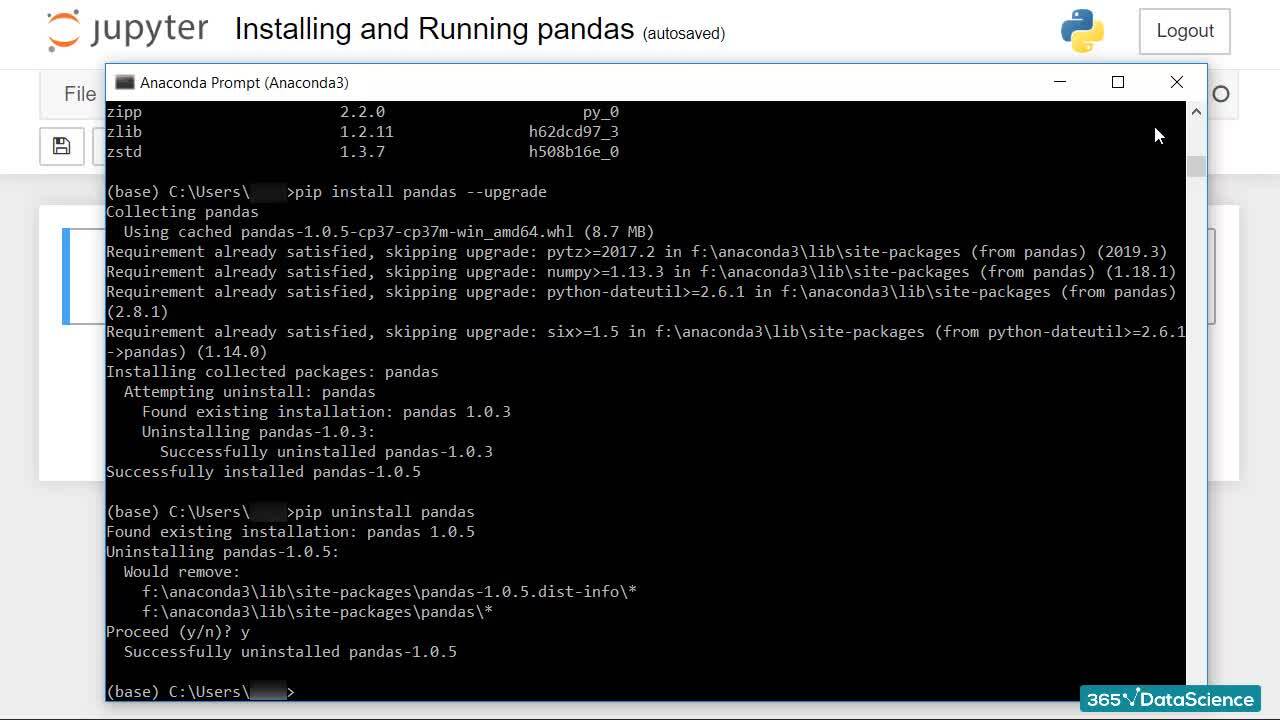
1.3 Installing and Running pandas
6 min
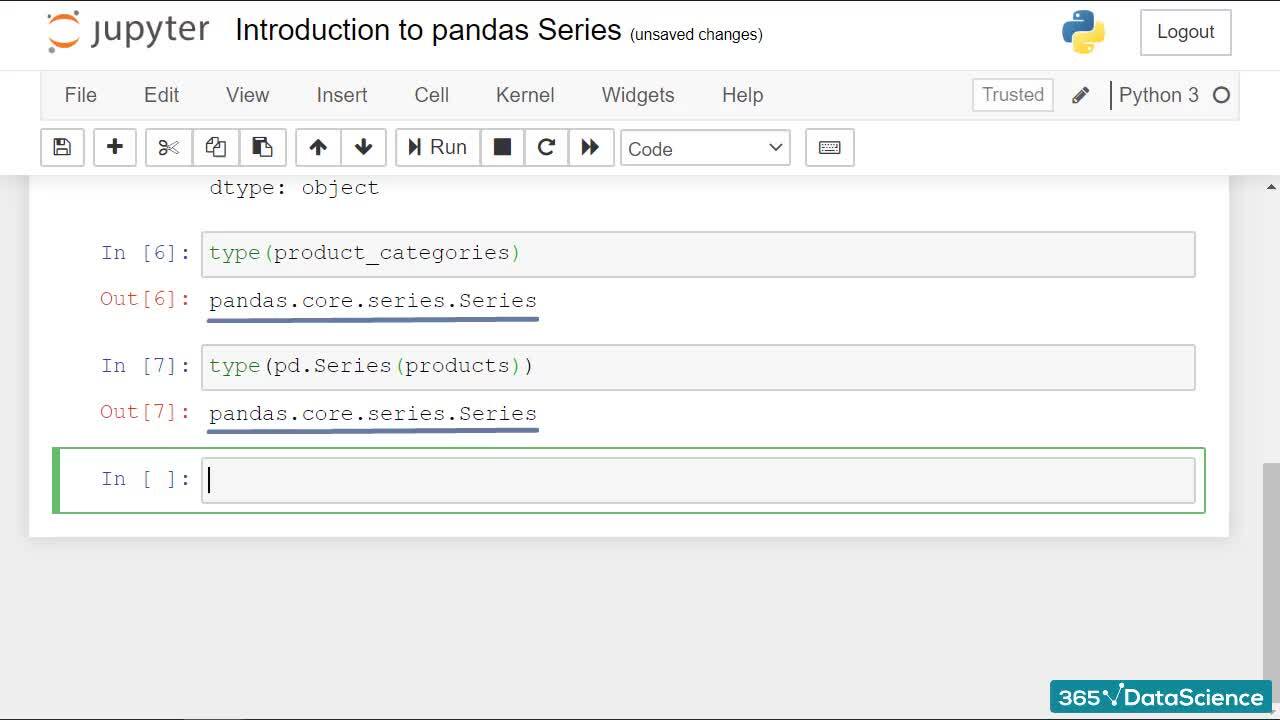
1.4 Introduction to pandas Series
9 min
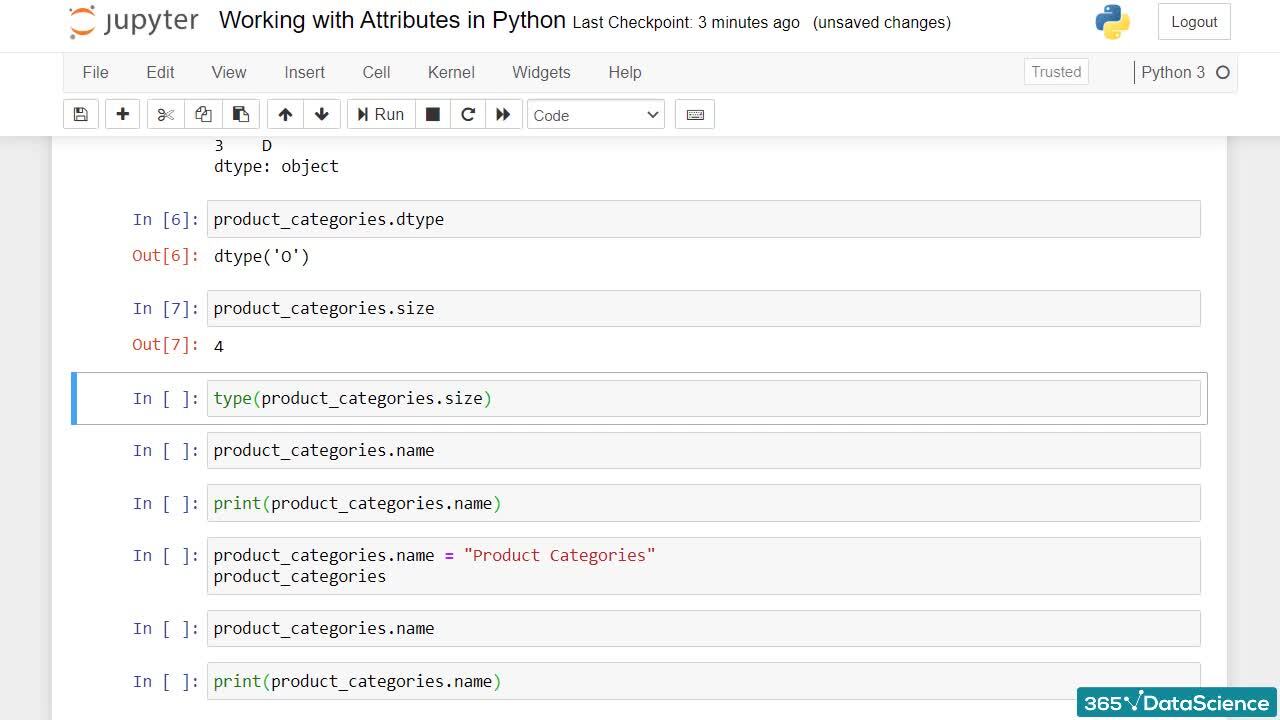
1.7 Working with Attributes in Python
5 min
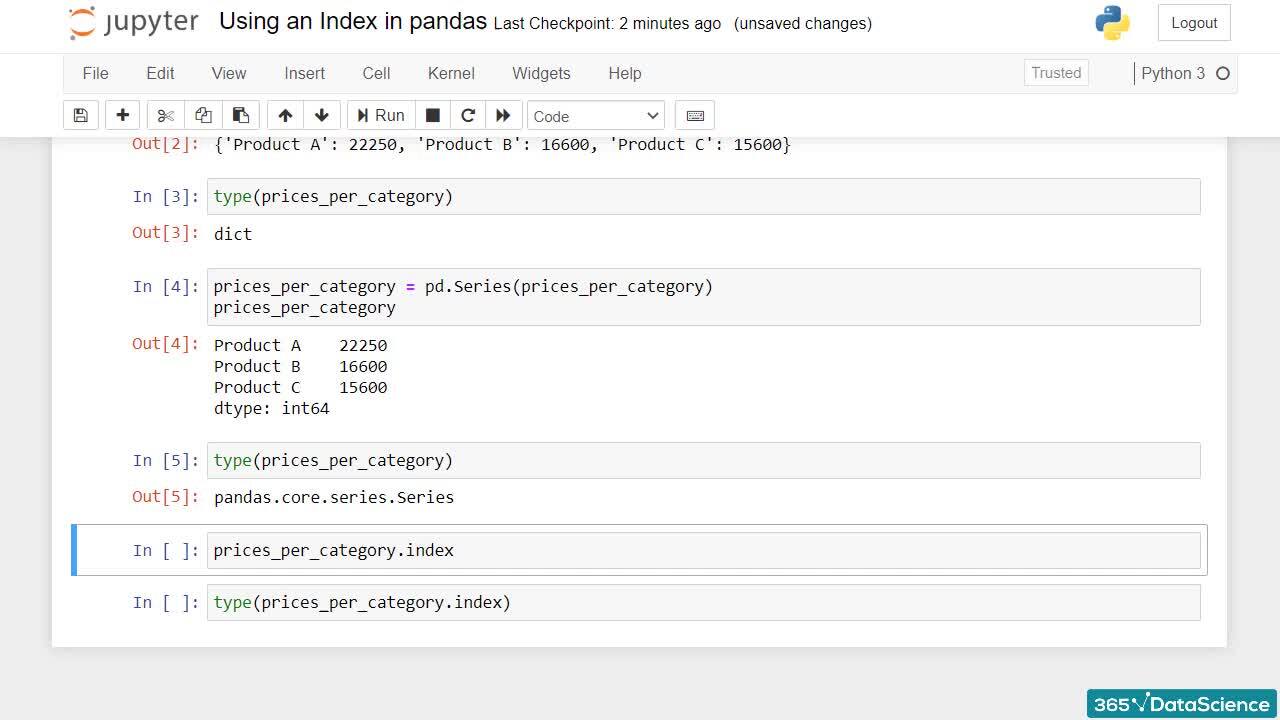
1.10 Using an Index in pandas
4 min
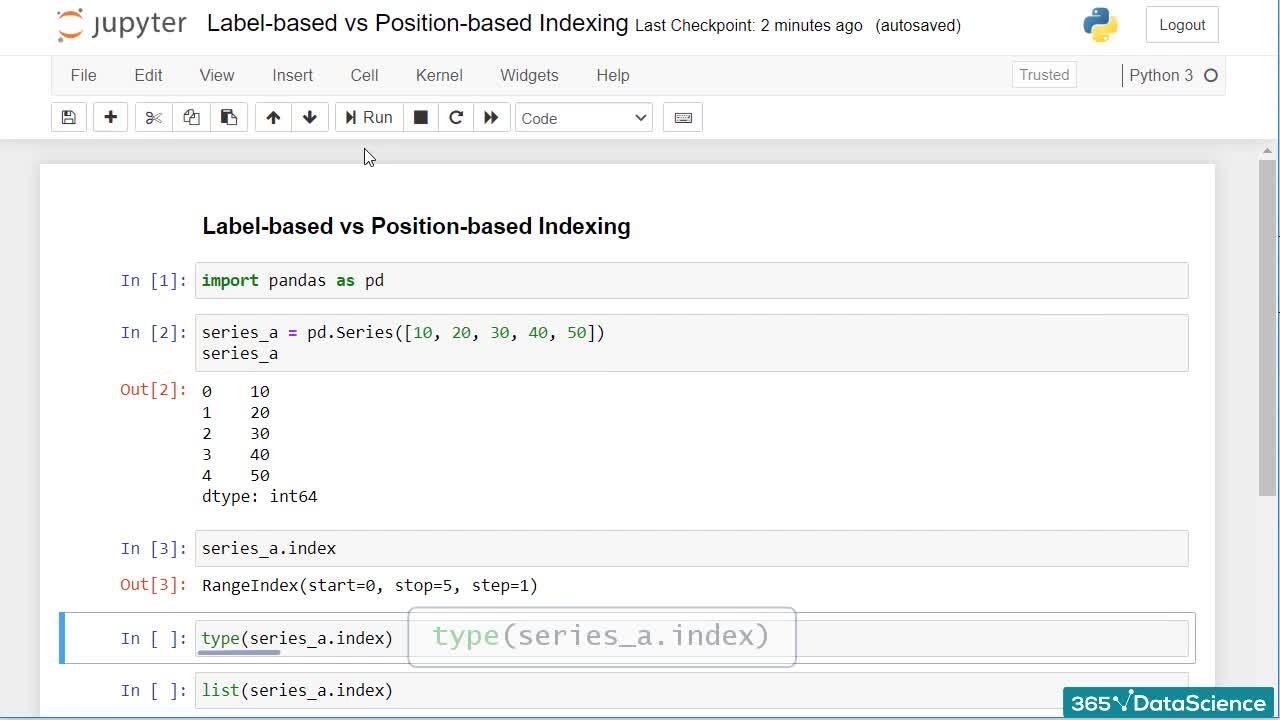
1.13 Label-based vs Position-based Indexing
5 min
Curriculum
- 2. Data Cleaning and Data Preprocessing1 Lesson 5 MinOnly about 20% of the work of a data analytics or science team goes to statistical analysis, making visualization or predictive models. The bulk of the time is consumed by collecting, cleaning, and preprocessing data. That is why in this section, we’ve provided a single lecture that aims at clarifying the meaning of and difference between the data cleaning and data preprocessing stages.Data Cleaning and Data Preprocessing5 min
- 3. pandas Series5 Lessons 21 MinHere, we will introduce you to working with one of the two core data structures of pandas – the pandas Series object. You will also discover several common methods and learn how to apply them to a pandas Series..unique(), .nunique()4 minConverting Series into Arrays5 min.sort_values()4 minAttribute and Method Chaining4 min.sort_index()4 min
- 4. pandas DataFrames8 Lessons 44 MinThis section focuses on the other fundamental object in pandas - the DataFrame. The DataFrame is universally known as the most important structure in this library. Here, we will revise its characteristics as well as comment on several popular related methods. In addition, we will show you how to deal with various techniques for data selection in a DataFrame.A Revision to pandas DataFrames5 minA Note on Working with the Anaconda Assistant Read now1 minUsing the Anaconda Assistant: Importing Data with pandas5 minCommon Attributes for Working with DataFrames4 minData Selection in pandas DataFrames7 minData Selection - Indexing Data with .iloc[]6 minData Selection - Indexing Data with .loc[]4 minA Few Comments on Using .loc[] and .iloc[]12 min
Topics
PythonData AnalysisProgrammingData PreprocessingPandasData PreprocessingProgramming
Course Requirements
- Highly recommended to take the Intro to Python course first
- You will need to install the Anaconda package, which includes Jupyter Notebook
Who Should Take This Course?
Level of difficulty: Intermediate
- Aspiring data analysts, data scientists, data engineers, AI engineers
- Graduate students who need Python and pandas for their studies
Exams and Certification
A 365 Data Science Course Certificate is an excellent addition to your LinkedIn profile—demonstrating your expertise and willingness to go the extra mile to accomplish your goals.

Meet Your Instructor
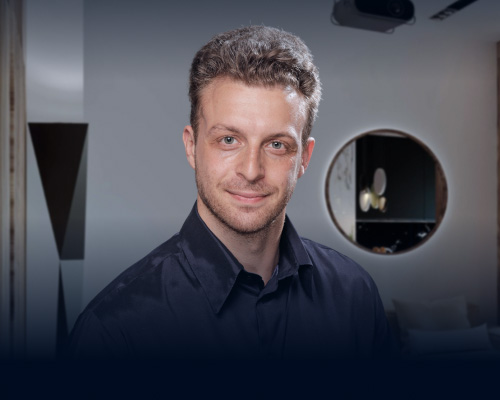
Martin began working with 365 in 2016 as the company’s second employee. Martin’s resilience, hard-working attitude, attention to detail, and excellent teaching style played an instrumental role in 365’s early days. He authored some of the firm’s most successful courses. And besides teaching, Martin dreams about becoming an actor. In September 2021, he enrolled in an acting school in Paris, France.
What Our Learners Say
365 Data Science Is Featured at
Our top-rated courses are trusted by business worldwide.