01.07.2025
Deep Learning with TensorFlow 2
with
Iskren Vankov
and
Iliya Valchanov
Master deep learning in Python with TensorFlow 2: Apply neural networks to solve real-world data science challenges
5 hours of content
17254 students
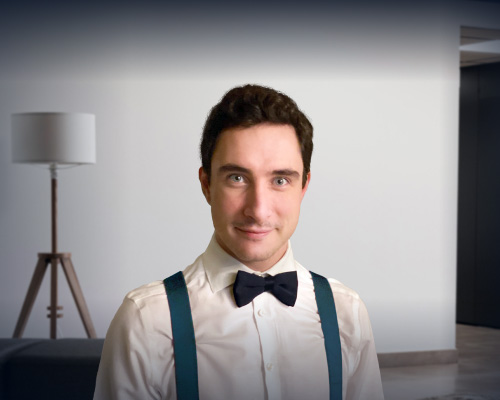
What you get:
- 5 hours of content
- 122 Interactive exercises
- 44 Downloadable resources
- World-class instructor
- Closed captions
- Q&A support
- Future course updates
- Course exam
- Certificate of achievement
Deep Learning with TensorFlow 2
A course by
Iskren Vankov
and
Iliya Valchanov
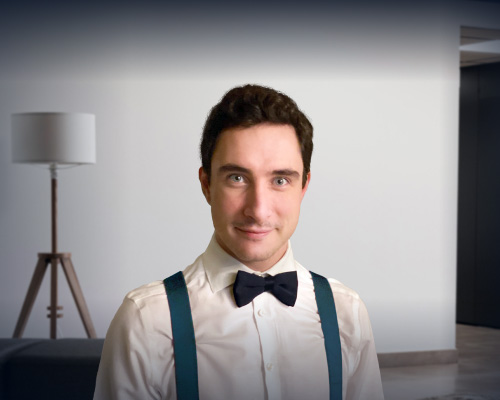
What you get:
- 5 hours of content
- 122 Interactive exercises
- 44 Downloadable resources
- World-class instructor
- Closed captions
- Q&A support
- Future course updates
- Course exam
- Certificate of achievement
$99.00
Lifetime access
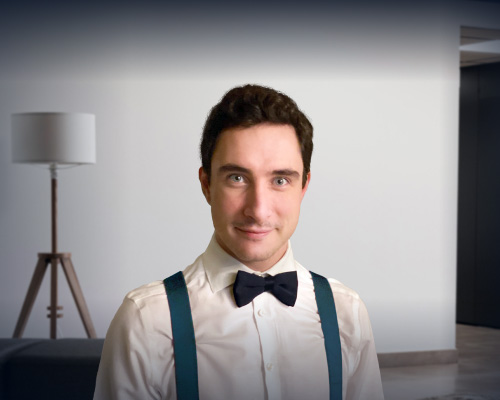
What you get:
- 5 hours of content
- 122 Interactive exercises
- 44 Downloadable resources
- World-class instructor
- Closed captions
- Q&A support
- Future course updates
- Course exam
- Certificate of achievement
What You Learn
- Master the essential mathematics for understanding deep learning algorithms
- Build and customize machine learning algorithms from scratch to enhance your control over model architecture
- Understand key deep learning concepts such as backpropagation, stochastic gradient descent, and batching to optimize your neural network models
- Learn how to deal with overfitting through early stopping and improve the generalizability of your models
- Solve complex real-world challenges in TensorFlow 2
- Improve your career perspectives by acquiring highly sophisticated technical skills such as deep learning in TensorFlow 2
- Position your profile to capitalize on the ever-growing number of AI development opportunities in the job market
Top Choice of Leading Companies Worldwide
Industry leaders and professionals globally rely on this top-rated course to enhance their skills.
Course Description
Machine and deep learning are some of those quantitative analysis skills that differentiate the data scientist from the other members of the team. Not to mention that the field of machine learning is the driving force of artificial intelligence. This course will teach you how to leverage deep learning and neural networks for the purposes of data science. The technology we employ is TensorFlow 2.0, which is the state-of-the-art deep learning framework.
Learn for Free
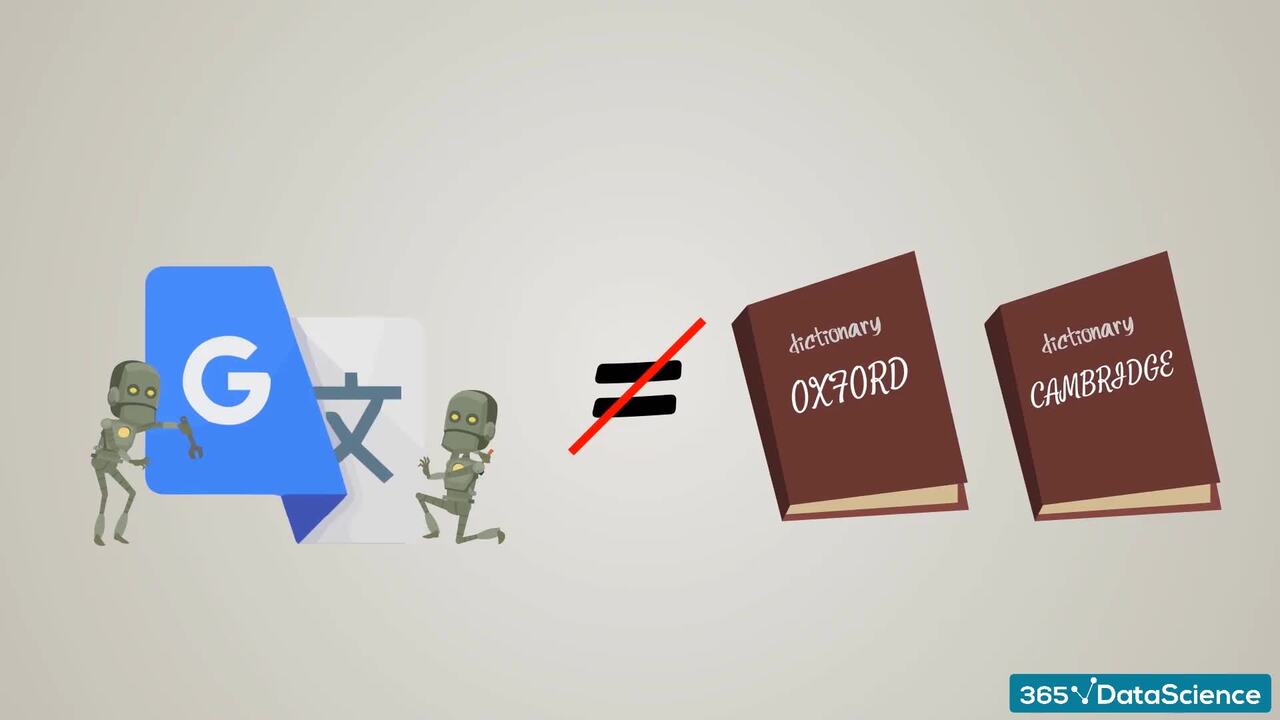
1.1 Why machine learning
7 min
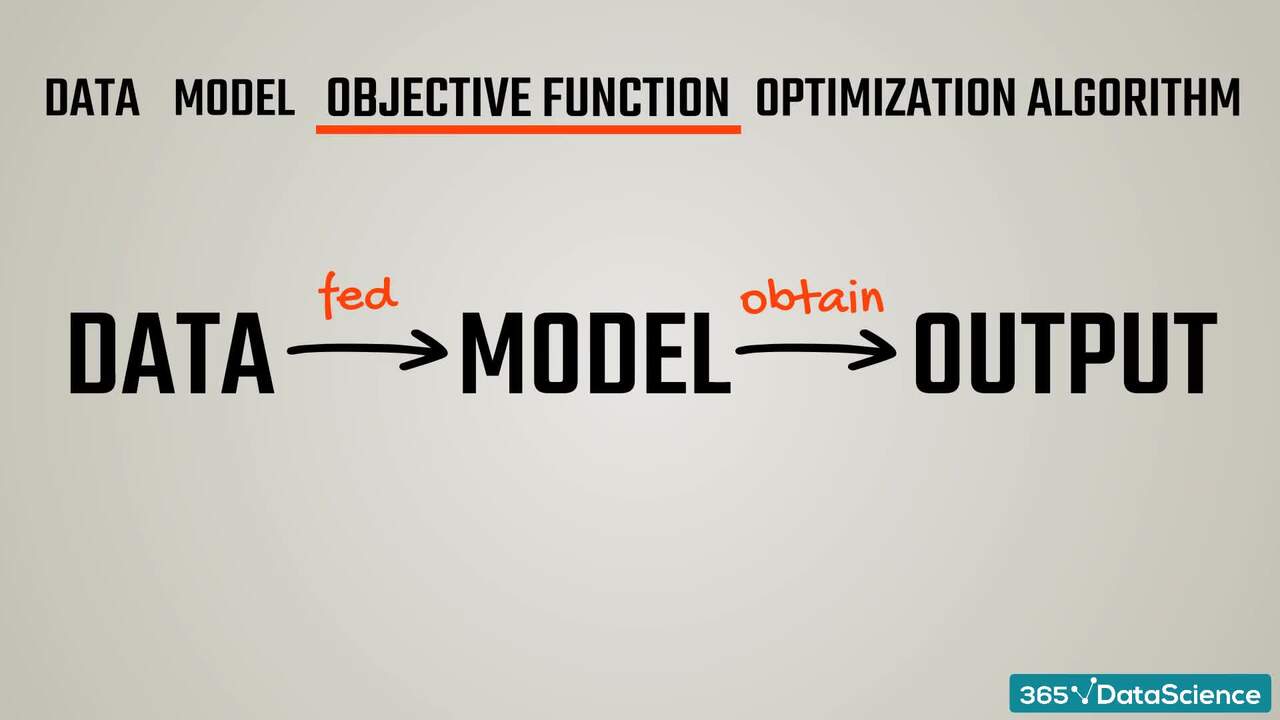
2.1 Introduction to neural networks
4 min
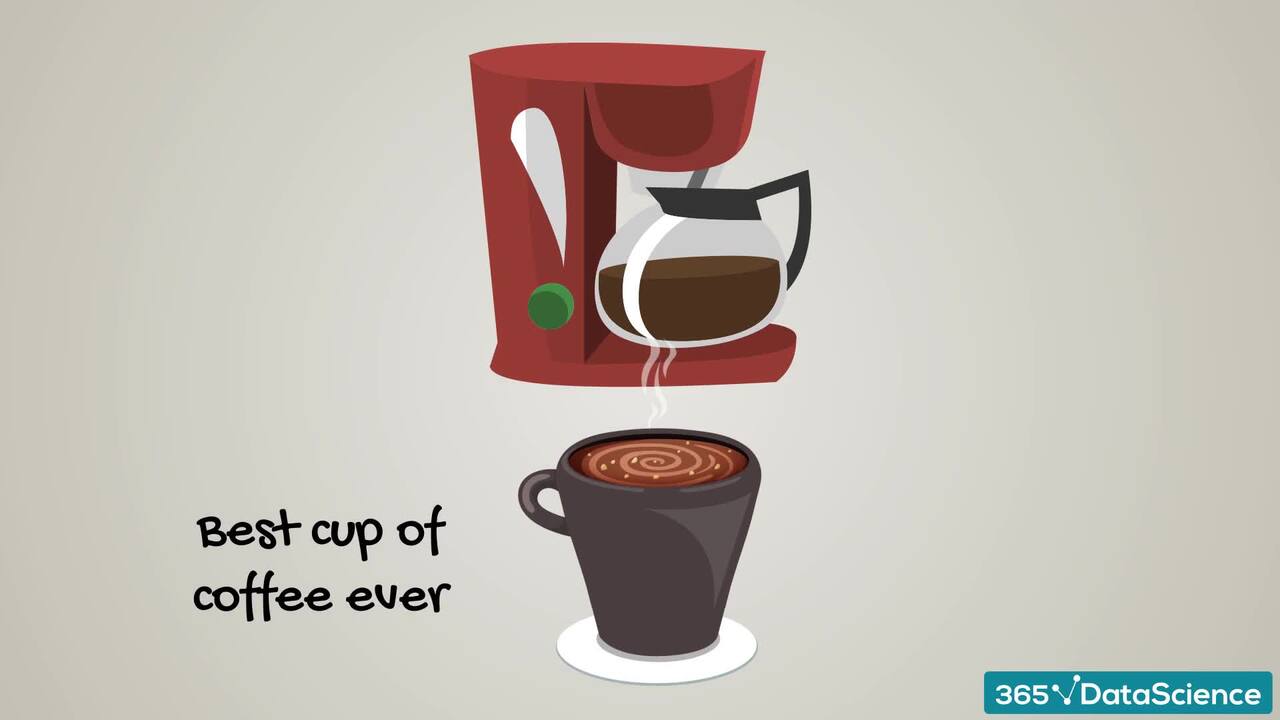
2.3 Training the model theory
3 min
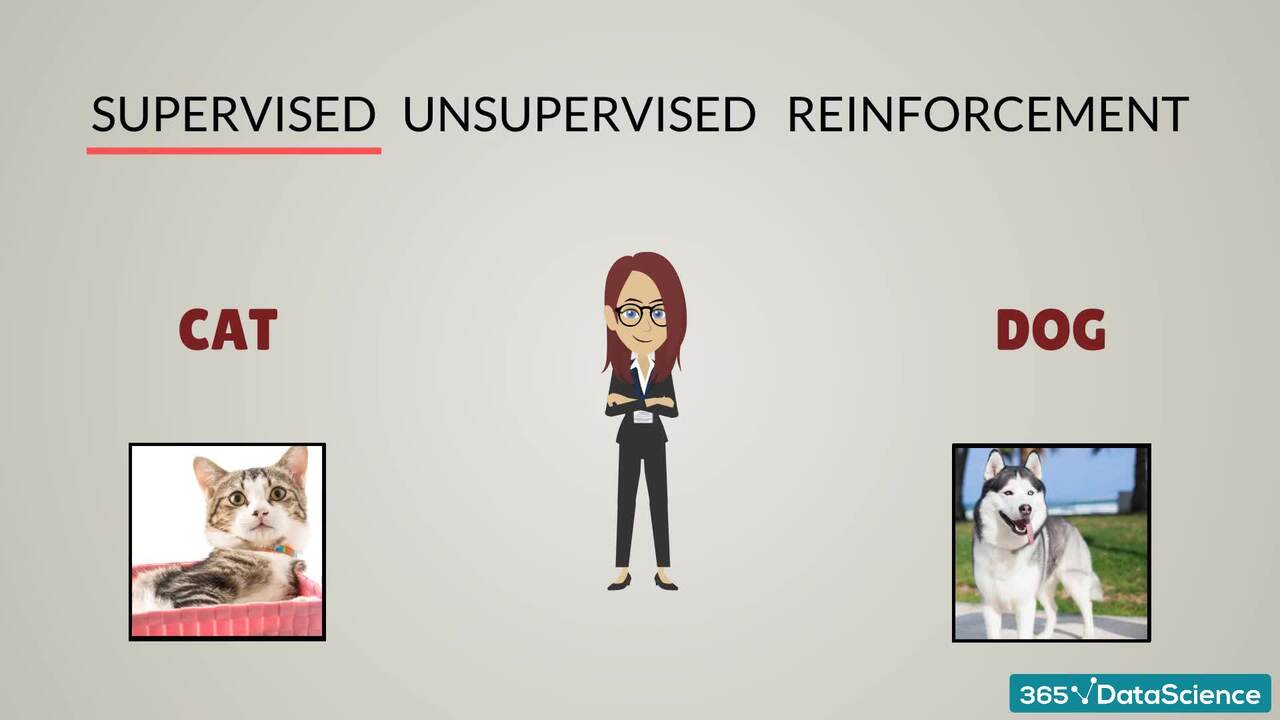
2.5 Types of machine learning
4 min
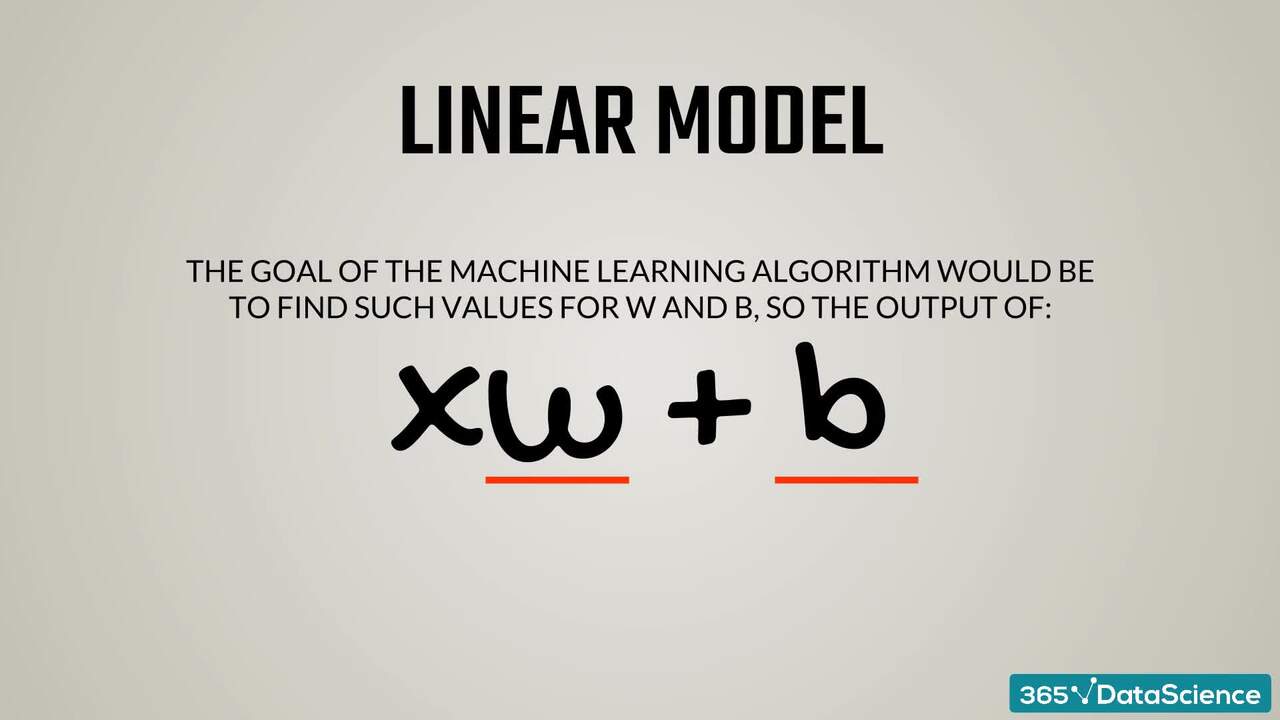
2.7 The linear model
3 min
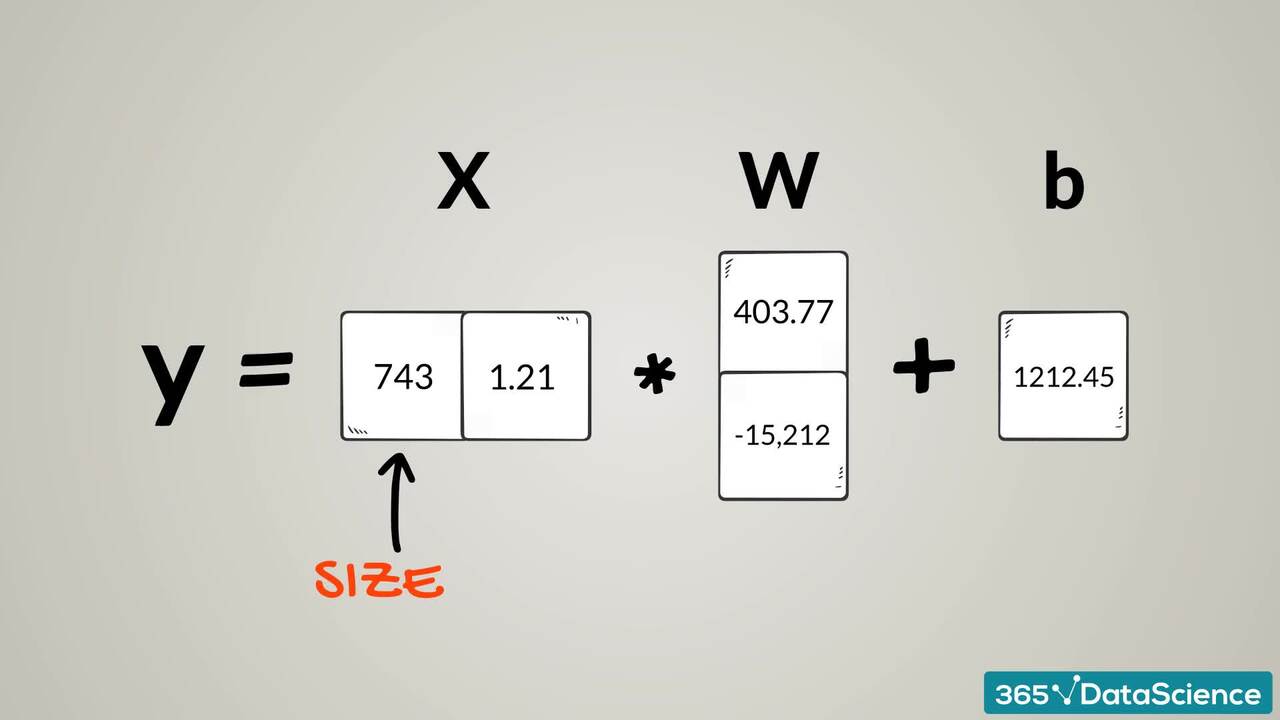
2.9 The linear model. Multiple inputs.
2 min
Curriculum
Topics
PythonTheoryMachine LearningProgrammingMathematicsDeep LearningTensorflowNeural NetworksMachine and Deep Learning
Course Requirements
- You need to complete an introduction to Python before taking this course
- Basic skills in statistics, probability, and linear algebra are required
- It is highly recommended to take the Machine Learning in Python course first
- You will need to install the Anaconda package, which includes Jupyter Notebook
Who Should Take This Course?
Level of difficulty: Advanced
- Aspiring data scientists, ML engineers, and AI developers
- Existing data scientists, ML engineers, and AI developers who want to improve their technical skills
Exams and Certification
A 365 Data Science Course Certificate is an excellent addition to your LinkedIn profile—demonstrating your expertise and willingness to go the extra mile to accomplish your goals.

Meet Your Instructor
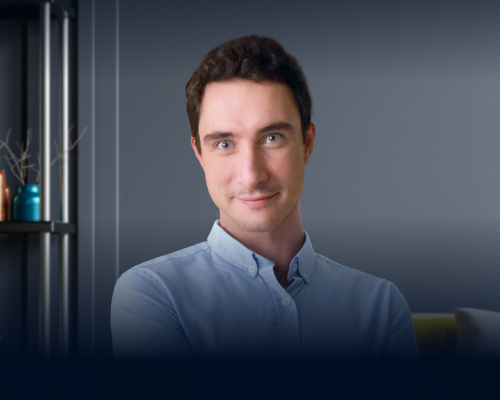
A top-of-class graduate from Edinburgh, Caltech, and Oxford, Iskren is a true academic force with over 8 years of industry experience, from Junior Developer to Head of Research and Development. He holds numerous prestigious awards, including the Microsoft Research Award for best Informatics dissertation and the BSc Computer Science and Physics Class Prize for the highest mark in his degree program. Iskren’s research background in Quantum Computation and experience in machine learning have contributed greatly to 365’s Deep Learning and CNNs courses, as well as the upcoming RNNs course.
What Our Learners Say
365 Data Science Is Featured at
Our top-rated courses are trusted by business worldwide.