23.06.2025
Product Management for AI & Data Science
trending topic
with
Danielle Thé
Grasp the full lifecycle of AI and data science projects: learn effective product management techniques
6 hours of content
10690 students
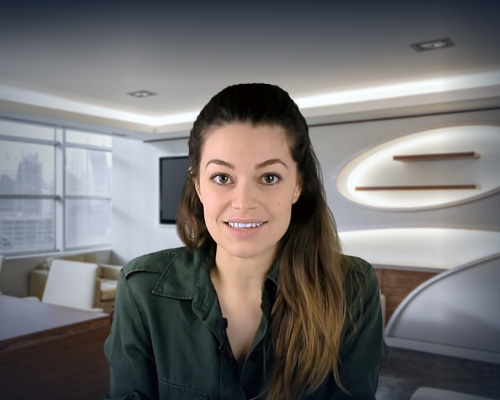
What you get:
- 6 hours of content
- 13 Interactive exercises
- 26 Downloadable resources
- World-class instructor
- Closed captions
- Q&A support
- Future course updates
- Course exam
- Certificate of achievement
Product Management for AI & Data Science
trending topic
A course by
Danielle Thé
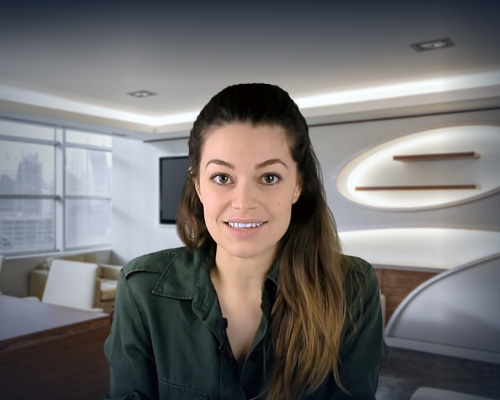
What you get:
- 6 hours of content
- 13 Interactive exercises
- 26 Downloadable resources
- World-class instructor
- Closed captions
- Q&A support
- Future course updates
- Course exam
- Certificate of achievement
$99.00
Lifetime access
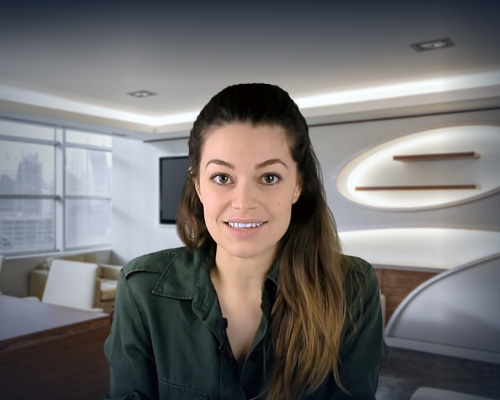
What you get:
- 6 hours of content
- 13 Interactive exercises
- 26 Downloadable resources
- World-class instructor
- Closed captions
- Q&A support
- Future course updates
- Course exam
- Certificate of achievement
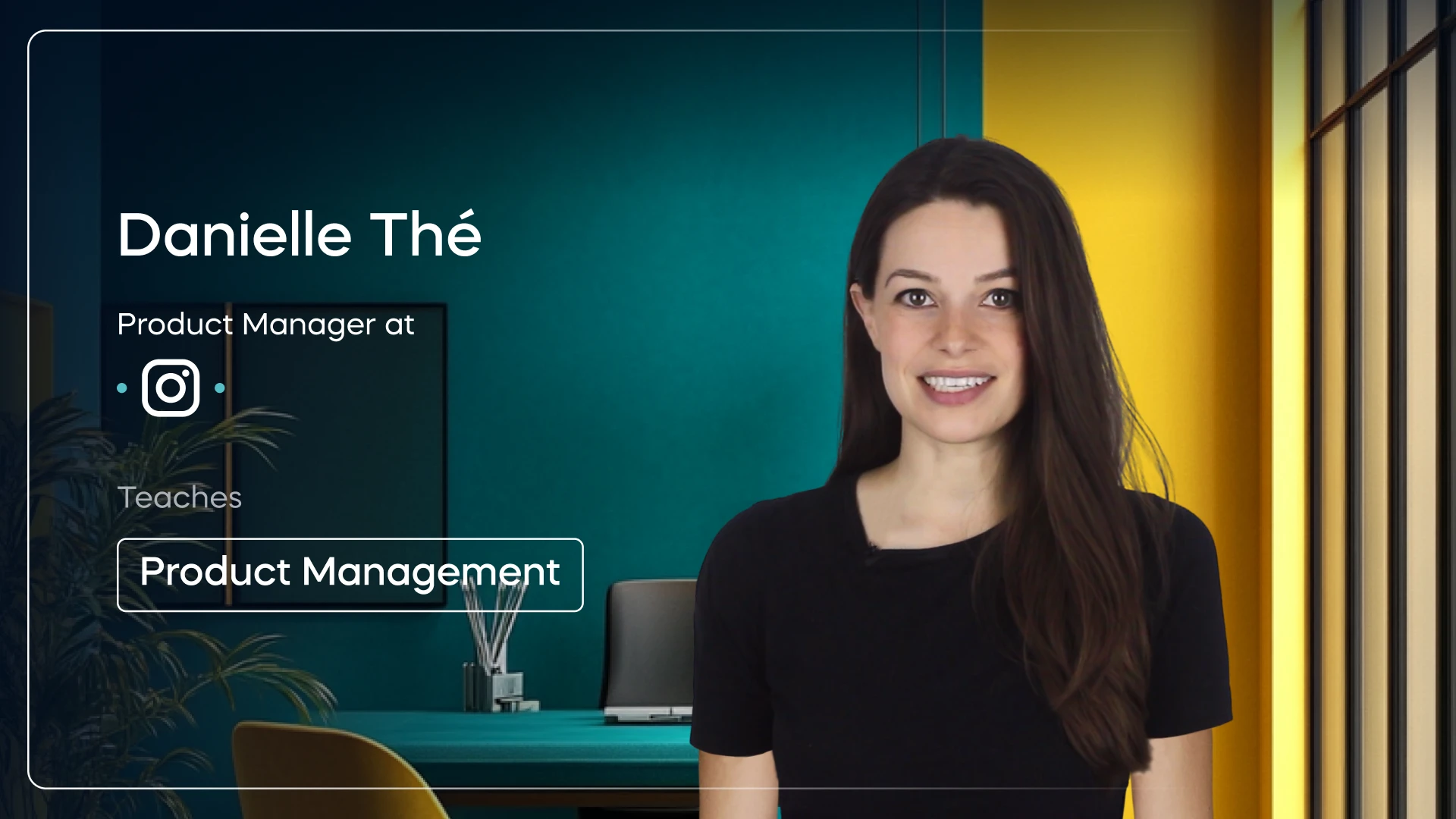
What You Learn
- Understand the responsibilities of a product manager in the context of data science and AI
- Improve communication between team members and stakeholders to foster effective collaboration
- Learn to strategically determine when to employ AI solutions in business operations to optimize resource allocation and avoid potential inefficiencies
- Gain a big-picture understanding of how data science and AI create business value
- Master essential technological concepts for data science and AI
- Differentiate your data scientist or AI Engineer profile by adopting a product manager’s perspective, enhancing your appeal to hiring managers
Top Choice of Leading Companies Worldwide
Industry leaders and professionals globally rely on this top-rated course to enhance their skills.
Course Description
This course is designed to teach you the specialized skills needed to manage the development of successful AI products. You'll learn how to identify opportunities for a business to leverage AI and prepare the data needed. In addition, you will grasp the organizational structure of AI and data science teams, how to communicate effectively with stakeholders, and the best methods for managing a team's workflow. After the course, you'll be able to take an idea, evaluate it with potential customers, prototype, strategize, build tests, and iterate on AI and data driven products.
Learn for Free
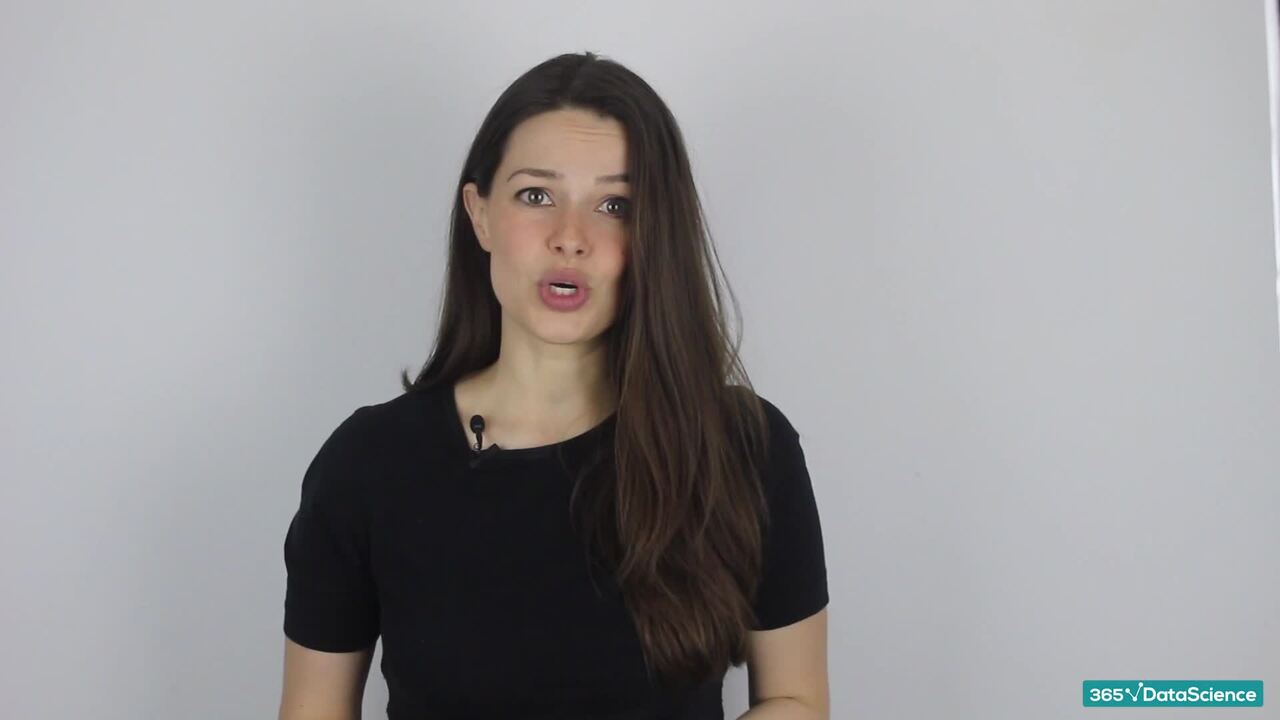
1.1 Introduction
4 min
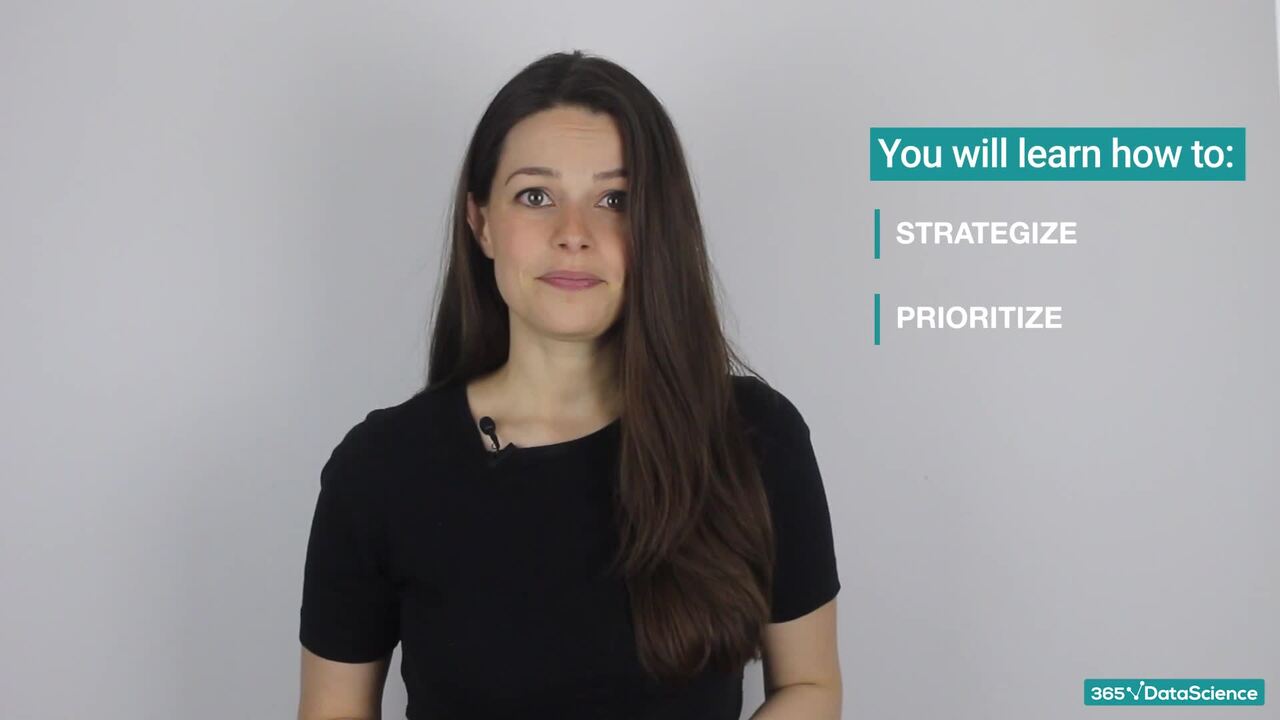
1.2 Course Overview
3 min
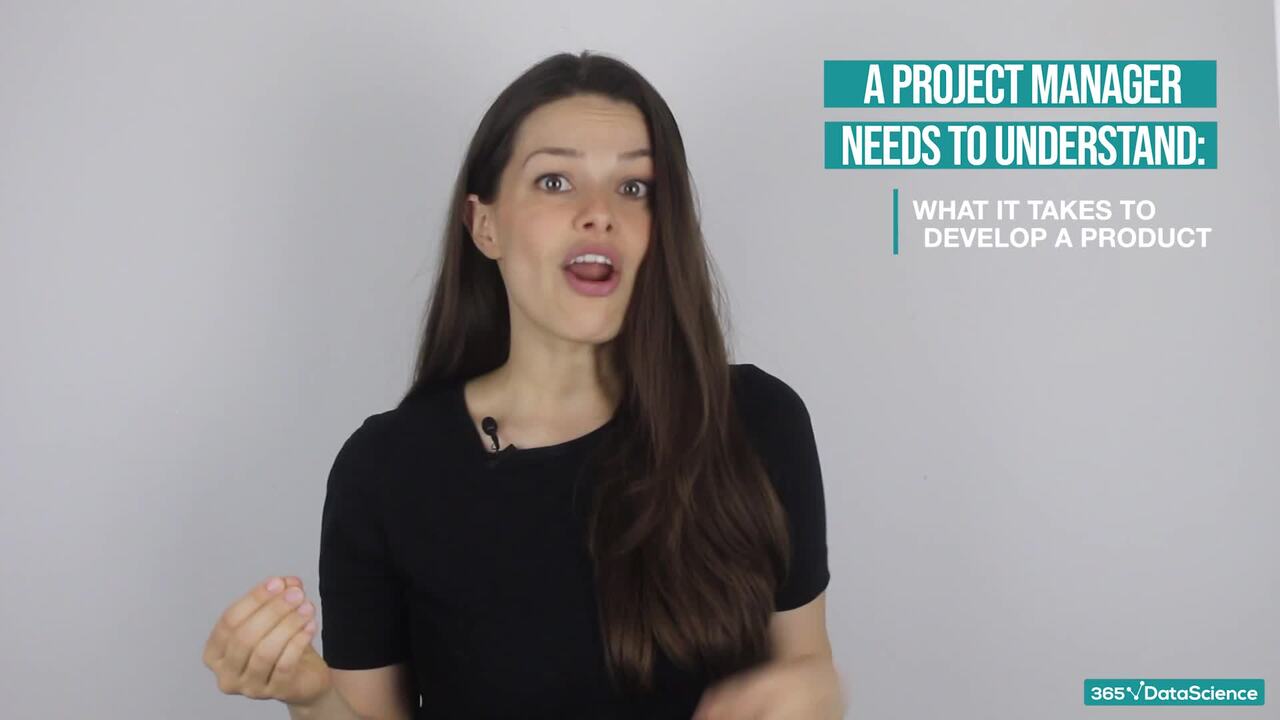
1.3 Growing Importance of an AI & Data PM
3 min
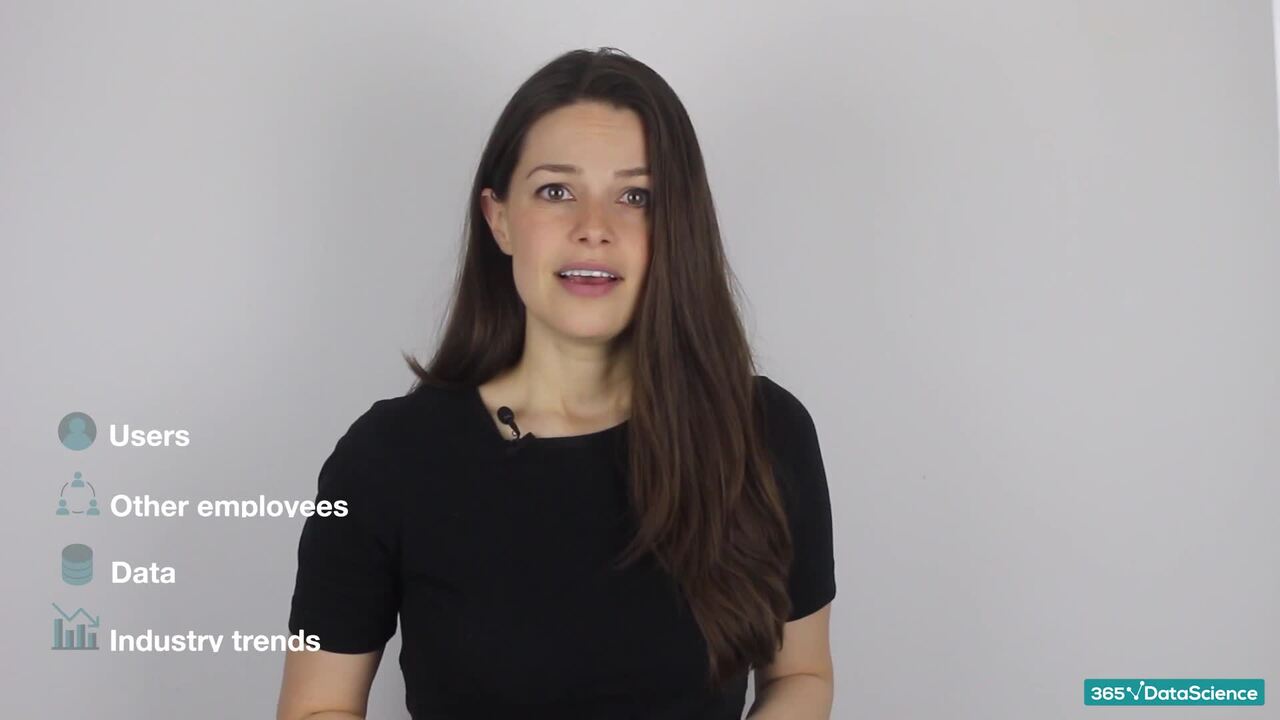
1.4 The Role of a Product Manager
5 min
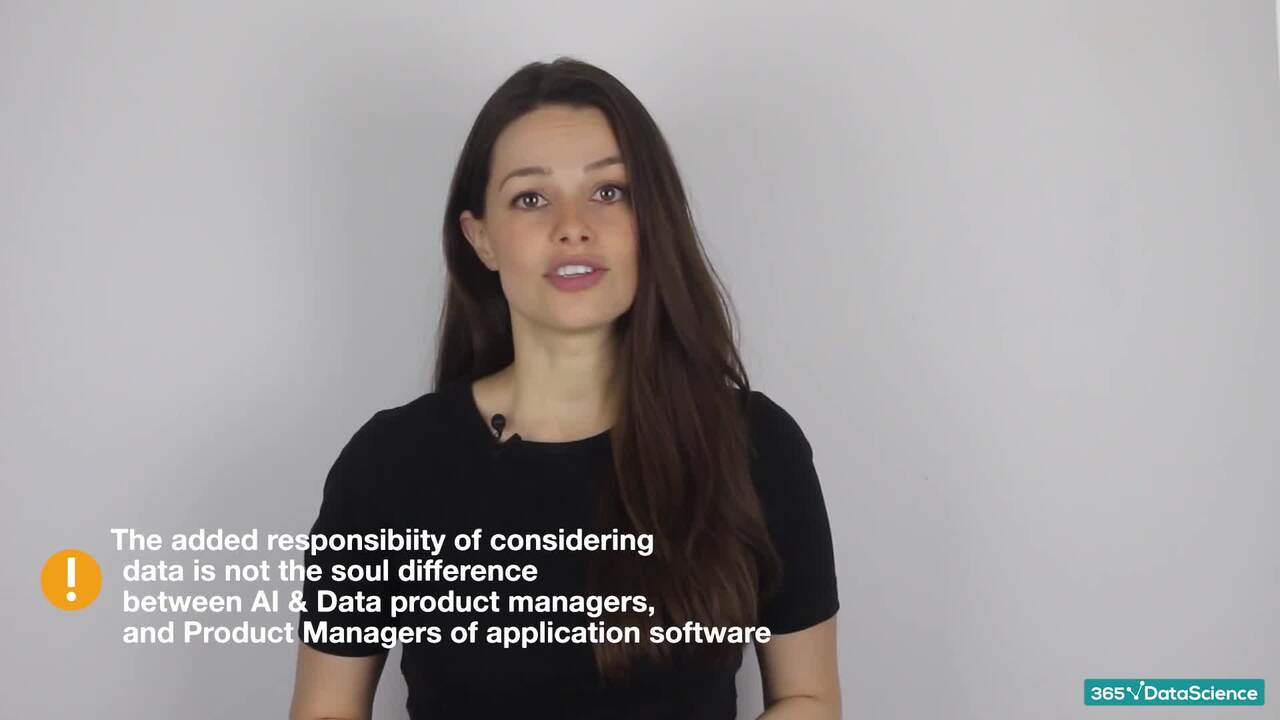
1.5 Differentiation of a PM in AI & Data
3 min
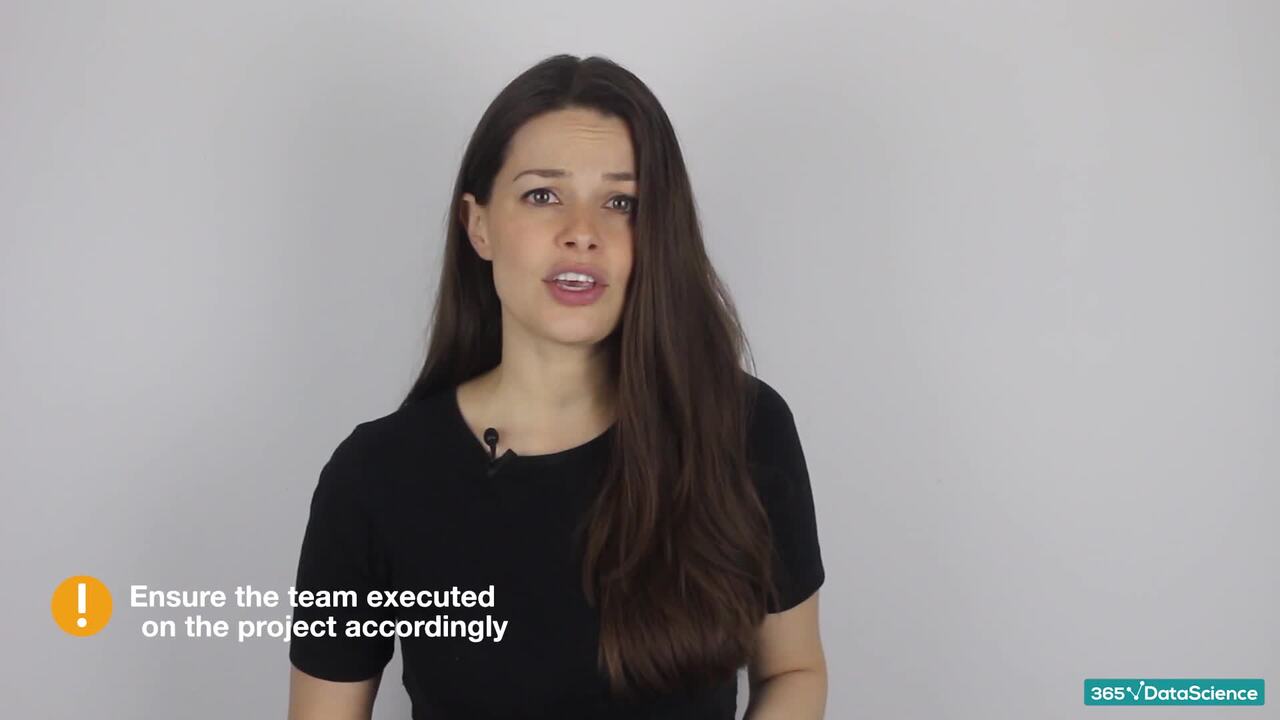
1.6 Product Management vs. Project Management
4 min
Curriculum
Topics
Product ManagementMachine LearningCareer DevelopmentUser ExperienceEthicsAITheoryData LiteracyAIBusiness Skills
Course Requirements
- No prior experience or knowledge is required. We will start from the basics and gradually build your understanding. Everything you need is included in the course
Who Should Take This Course?
Level of difficulty: Beginner
- Aspiring business analysts, data analysts, and data scientists
- Current business analysts, data analysts and data scientists who want to boost their business acumen and product management skills
- Managers in small, mid-sized, and large organizations
Exams and Certification
A 365 Data Science Course Certificate is an excellent addition to your LinkedIn profile—demonstrating your expertise and willingness to go the extra mile to accomplish your goals.

Meet Your Instructor
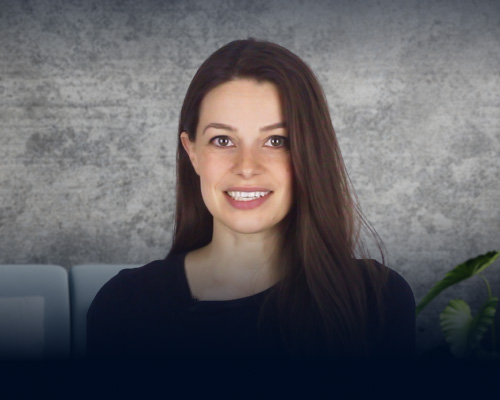
Danielle’s expertise in product marketing, product management, and project management is beyond impressive. She has worked with two of the FAANG companies. At Google, Danielle was involved in various initiatives for the YouTube brand, where she continued to develop her professional skills. Her extensive experience includes working with Data Scientists to leverage Natural Language Processing in storytelling for Wattpad, as well as founding Developers Without Borders - an online platform that connects software developers worldwide with development projects. Danielle describes herself as “a tech enthusiast that makes the digital world easy to understand”. Now and then, Danielle posts interesting videos on her YouTube channel. Her content is extremely engaging and fun to watch, so we definitely recommend checking it out.
What Our Learners Say
365 Data Science Is Featured at
Our top-rated courses are trusted by business worldwide.